Contents of this page is copied directly from AWS blog sites to make it Kindle friendly. Some styles & sections from these pages are removed to render this properly in 'Article Mode' of Kindle e-Reader browser. All the contents of this page is property of AWS.
Page 1|Page 2|Page 3|Page 4
The Most Viewed APN Blog Posts in 2021
=======================
From a wide range of AWS Partner success stories to new partner programs launched at AWS re:Invent, here’s a look at the most popular APN Blog posts we shared in 2021.
Our goal with this blog has always been to share timely and relevant news, technical solutions, partner stories, and more from Amazon Web Services (AWS) and the AWS Partner Network (APN) specifically.
The APN is a global community of partners that leverages programs, expertise, and resources to build, market, and sell customer offerings. Together, partners and AWS can provide innovative solutions, solve technical challenges, win deals, and deliver value to our mutual customers.
For customers, the APN helps you identify companies with deep expertise on AWS that can deliver on core business objectives. The robust AWS Partner community features over 100,000 partners from more than 150 countries. This vibrant, diverse network of partners can help you drive greater value for your business, increase agility, and lower costs.
Thank you for reading the APN Blog, and Happy New Year to all our AWS Partners and customers!
Top Partner Success Posts of the Year
These were the most viewed APN Blog posts published in 2021 that focused on AWS Partner successes and how-tos:
- Deploying IBM Mainframe z/OS on AWS with IBM ZD&T
- Mainframe Data Migration to AWS with Infosys (guest post by Infosys)
- Palantir Foundry Helps You Build and Deploy AI-Powered Decision-Making Applications (guest post by Palantir)
- Amazon S3 Malware Scanning Using Trend Micro Cloud One and AWS Security Hub (guest post by Trend Micro)
- Design Considerations for Disaster Recovery with VMware Cloud on AWS
- Taming Machine Learning on AWS with MLOps: A Reference Architecture (guest post by Reply)
- How to Get Logs from Amazon S3 Using Filebeat and Metricbeat in Elastic Stack (guest post by Elastic)
- Implementing Multi-Factor Authentication in React Using Auth0 and AWS Amplify
- Amazon AppFlow for Bi-Directional Sync Between Salesforce / Amazon RDS for PostgreSQL (guest post by Trantor)
- Data Tokenization with Amazon Redshift and Protegrity (guest post by Protegrity)
Read all of our AWS Partner success stories >>
Top Partner Program Posts of the Year
These posts were the most viewed APN Blog posts in 2021 about our AWS partner programs:
- 2021 Japan APN Ambassadors / 2021 APN Top Engineers
- Architecting Successful SaaS: Understanding Cloud-Based Software-as-a-Service Models
- Building a Multi-Tenant SaaS Solution Using Amazon EKS
- Announcing the AWS Partner Network’s Regional 2021 Partners of the Year
- AWS Partner Paths: A New Way to Accelerate Engagement with AWS
- AWS Mainframe Migration Competency Featuring Validated AWS Partners
- Introducing New AWS Solution Provider Program Incentives for AWS Partners
- AWS Control Tower Best Practices for AWS Solution Providers
- Getting Out of Your Own Way: How to Avoid Common SaaS Pitfalls
- Introducing the AWS Energy Competency Partners
Read all of our APN program posts >>
Top All-Time Posts of the Year
These posts that we have published since the APN Blog’s inception were the most viewed in 2021:
- The 5 Pillars of the AWS Well-Architected Framework
- Terraform: Beyond the Basics with AWS
- Using Terraform to Manage AWS Programmable Infrastructures (guest post by Cloudsoft)
- Now You Can Take the AWS Certified Cloud Practitioner Exam at Your Home or Office 24/7
- AWS Lambda Custom Runtime for PHP: A Practical Example (guest post by Rackspace)
- Making Application Failover Seamless by Failing Over Your Private Virtual IP Across Availability Zones
- Connecting AWS and Salesforce Enables Enterprises to Do More with Customer Data
- How to Get AWS Certified: Tips from a DevOps Engineer (guest post by SQUADEX)
- Using GitLab CI/CD Pipeline to Deploy AWS SAM Applications
- Getting Started with Ansible and Dynamic Amazon EC2 Inventory Management
Bookmark the APN Blog for the latest updates >>
Say Hello to New AWS Partners Added in 2021
Each month on the APN Blog, we highlight AWS Partners that received new designations for our global AWS Competency, AWS Managed Service Provider (MSP), AWS Service Delivery, and AWS Service Ready programs.
These designations span workload, solution, and industry, and help AWS customers identify top AWS Partners that can deliver on core business objectives.
See the running list of new AWS Partners >>
Stay Connected
Follow the AWS Partner Network (APN) on social media for all the latest updates:
Follow @AWS_Partners on Twitter
Join the Scale with AWS Partners page on LinkedIn
Subscribe to the AWS Partner YouTube channel
Join the AWS Partner email list for timely updates about APN news and events
AWS Partner Paths: A New Way to Accelerate Engagement with AWS
=======================
By Priya Bains, Sr. Product Manager – AWS Partner Network
By Christine Linthacum, Principal Product Manager – AWS Partner Network
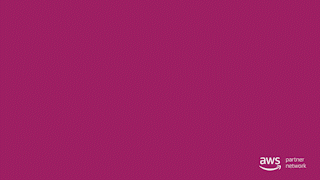
We are excited to introduce new AWS Partner Paths, a flexible way to accelerate engagement with Amazon Web Services (AWS) starting January 28, 2022.
Partners have shared with us that they need more ways to work with AWS faster as their business evolves to meet customer needs—Partner Paths are a response to this evolution.
This framework provides a curated journey through partner resources, benefits, and programs. Partner Paths replace technology and consulting partner type models—evolving to an offering type model.
Last year, we launched the first ISV Partner Path. The new Paths support more customer offerings and provide partners flexibility in all the ways they engage with AWS. We now offer five Partner Paths—Software Path, Hardware Path, Training Path, Distribution Path, and Services Path—which represents consulting, professional, managed, or value-add resale services.
Partners can enroll in one or multiple Paths based on their unique customer offerings.
As part of this announcement, we are also renaming the ISV Path to Software Path to better reflect offering types, rather than partner types.
AWS Partner Paths simplify the engagement model for partners, expand partner access to benefits, and provide a more intuitive and streamlined experience.
Simplified Engagement
AWS Partner Paths are purpose-built to support and strengthen a range of partners’ customer offerings. We provide organizations that register with the AWS Partner Network (APN) access to a dedicated partner portal, training discounts, business and technical enablement content, programs, and benefits relevant to their offerings.
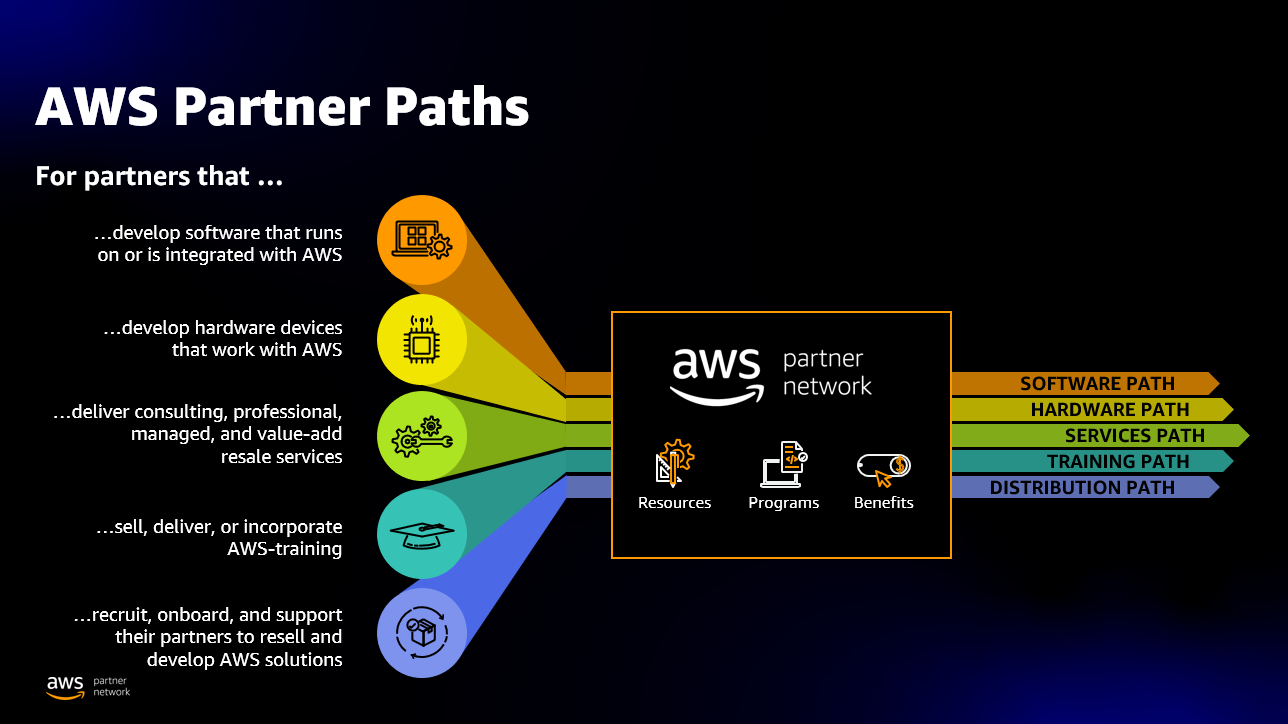
Expanding Access to Partner Benefits
As AWS Partners progress along each Path, they can leverage enablement resources, unlock funding benefits, and tap into a broad set of programs to innovate, expand, and differentiate their customer offerings.
There are no tier requirements to validate software and hardware offerings with AWS. Partners can earn a qualified software and/or hardware badge, and directly access several AWS resources specifically designed for the Software or Hardware Paths.
For organizations engaged with the Services and Training Path—tiers continue to remain important to customers to understand the depth of their experience.
For example, a partner may enroll in the Software Path and utilize the AWS SaaS Factory program to help optimize their solution and complete a Foundational Technical Review (FTR) to achieve the “AWS Qualified Software” badge for their offering, while also enrolling in the Services Path to achieve partner tier recognition for their AWS-aligned professional capabilities and managed services experience.
We also have a dedicated Partner Path for distribution, an invitation-only Path to help Authorized Distributors engage faster with AWS.
Intuitive Experience for Partners
In support of this new framework, we have streamlined the AWS Partner Central experience to empower partners with the right tools that make it easier to self-serve.
This new user experience—another response to partner feedback—will help AWS Partners navigate through enablement resources, structured content, benefits, and programs to help them better showcase customer expertise.
As with everything we do at AWS, customer obsession is a core part of our business. We’re obsessed with giving our partners the flexibility and agility to thrive in the business areas of their choice, and we look forward to rolling out these Partner Paths in early 2022.
Get Started
Learn more about the new AWS Partner Paths.
Join the AWS Partner Network (APN) to build, market, and sell with AWS.
Gaining Critical Security Insights and Control of Your Traffic with Aviatrix ThreatIQ and ThreatGuard
=======================
By James Devine, VP Product Management – Aviatrix
By Jacob Cherkas, Principal Architect – Aviatrix
By Mandar Alankar, Sr. Networking Solutions Architect – AWS
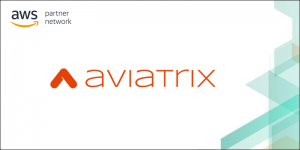 |
Aviatrix |
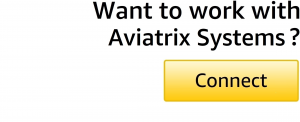 |
It can be difficult to gain security insights into your cloud infrastructure, especially as architectures grow to encompass multiple availability zones, regions, and clouds.
Aviatrix Systems is an AWS Partner with Competency designations in both Networking and Security. Aviatrix is uniquely positioned to provide deep insights into network traffic.
This includes security insights that can augment Amazon Web Services (AWS) native security capabilities, such as those found by Amazon GuardDuty, for example.
In a previous blog post, we discussed the advanced visibility and troubleshooting capabilities that Aviatrix brings to AWS customers. In this post, we’re excited to detail new capabilities that were recently added to the Aviatrix Secure Network Platform—ThreatIQ and ThreatGuard.
Threat Detection with ThreatIQ
Aviatrix ThreatIQ is a capability that detects malicious traffic based on IP address reputation and known bad actors. It serves as an intrusion detection system (IDS) to alert on malicious traffic throughout your cloud architecture.
ThreatIQ uses a threat feed of known malicious IP addresses that is continuously updated. This ensures you always have an up-to-date detection capability.
Since Aviatrix Gateways export data flow summaries as NetFlow, it’s possible to have a view of threats across an entire AWS infrastructure as well as external connections and clouds.
Alerts are aggregated from all Aviatrix Gateways within and across regions, and even other clouds. This capability embeds detection inside the data plane, rather than limiting threat visibility to the edge of the network.
All traffic is analyzed in real-time as it goes across the Aviatrix Platform and becomes visible in the Aviatrix CoPilot web console.
Configuring an overly permissive security group on an Amazon Elastic Compute Cloud (Amazon EC2) instance quickly shows the power of ThreatIQ. Aviatrix has done just that and left the instance running; as you can see below, a holistic view of all the threats that were detected.
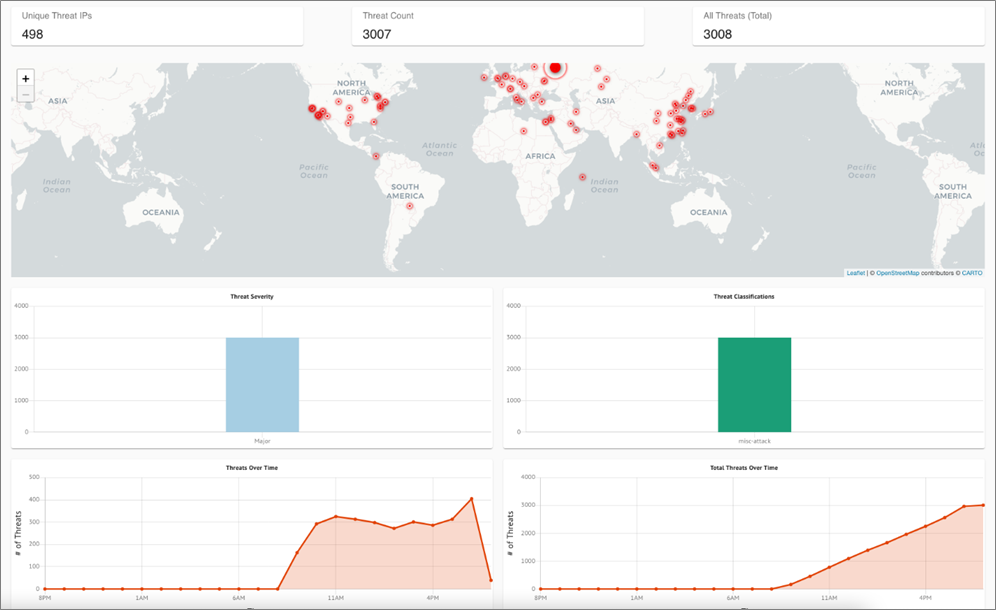
Figure 1 – ThreatIQ dashboard.
You can also view a line-by-line summary of detected threats. This allows you to drill down into individual findings to get more details, including access to raw NetFlow data and topology information.
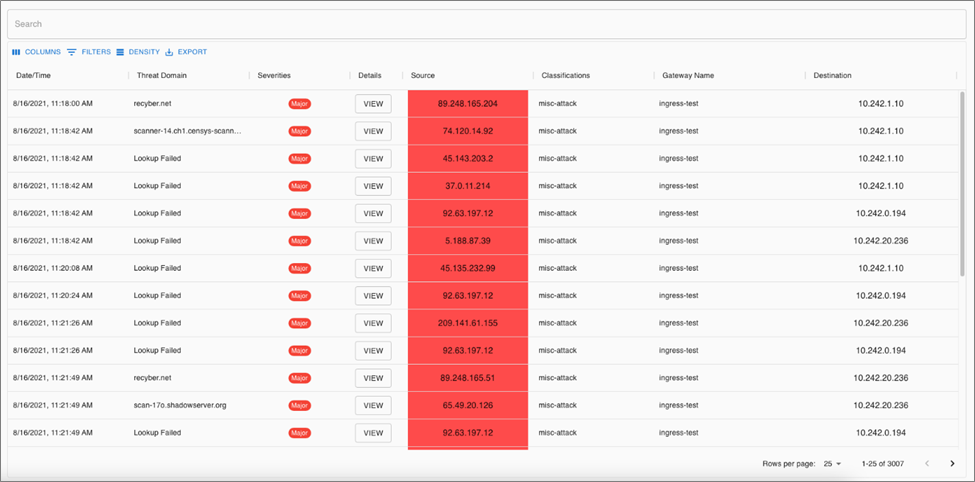
Figure 2 – ThreatIQ threat details.
Each of the threats can be further examined to see exactly where in the environment each finding was detected.
Such a view is quite difficult to get from traditional tools that don’t have an end-to-end topology view and understanding of your cloud network. This provides an invaluable tool for incident response to allow security teams to pinpoint the exact source of a malicious traffic flow. Root cause analysis becomes a breeze.
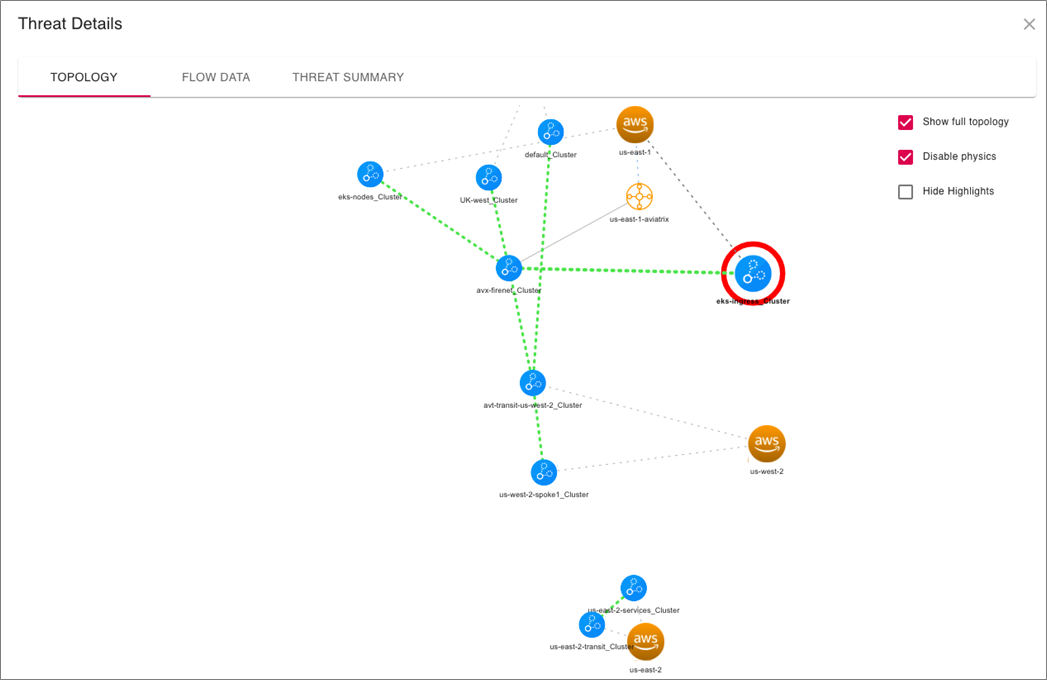
Figure 3 – ThreatIQ threat details.
The capability doesn’t stop there. You can double-click on the Aviatrix Gateway to drill all the way down to the individual instance that is the source/destination of the identified malicious traffic. This powerful capability is difficult to impossible to accomplish without Aviatrix.
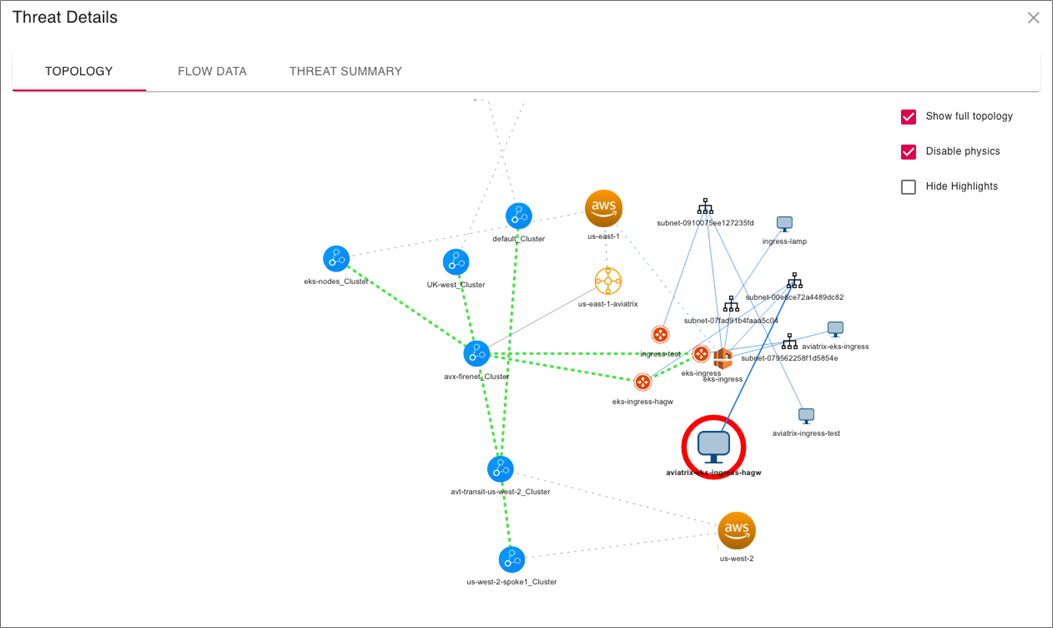
Figure 4 – ThreatIQ instance view.
Remediation with ThreatGuard
ThreatIQ provides a compelling IDS capability that augments Amazon GuardDuty findings; however, the Aviatrix platform takes these capabilities further.
You can enable automatic remediation to turn the IDS capability into an intrusion prevention system (IPS). This feature, called ThreatGuard, takes the ThreatIQ findings and programs deny rules in Aviatrix Gateways to block the traffic at the source virtual private cloud (VPC) of the identified host.
It can also send webhooks to external services like Slack and email notifications so your Security Operations Center (SOC) gets notified as soon as malicious traffic is detected.
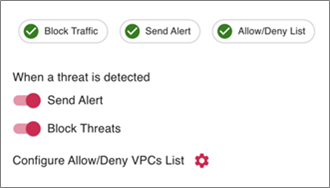
Figure 5 – Enabling ThreatGuard.
Once enabled, you see the stateful firewall rules that get programmed on Aviatrix Gateways to block malicious traffic. Below, you can see where stateful firewall rules have been programmed into Aviatrix Gateways to block malicious traffic flows.
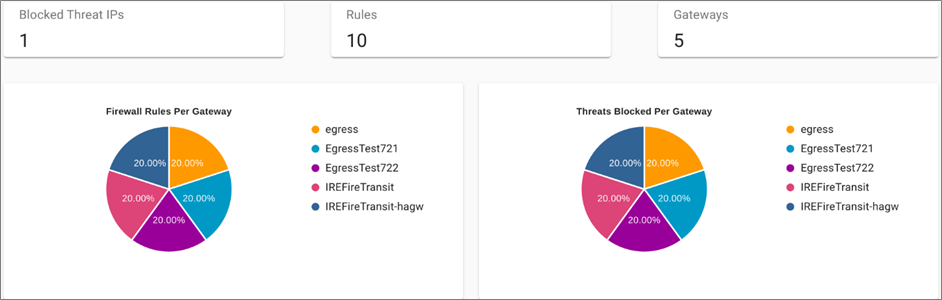
Figure 6 – ThreatGuard block rules.
Customer Benefits
Aviatrix enables you to deploy secure-by-default, best-practice network architectures. This enables networking teams to easily codify and provision repeatable network architectures that security teams have full flow-level visibility into.
Application developers and application owners have full autonomy to deploy their application within the guardrails that the Aviatrix Secure Networking Platform provides.
Aviatrix is already helping customers detect, identify, and remediate malicious traffic flows in their cloud deployments. Even customers that thought they had a strong security perimeter are discovering malicious traffic patterns using ThreatIQ.
Conclusion
In this post, we discussed ThreatIQ with ThreatGuard and how each can help you augment cloud-native capabilities.
In addition to the Aviatrix Secure Networking Platform bringing a single-tenant data plane to AWS, it also serve as a distributed security inspection and remediation mechanism. With end-to-end control and visibility, the network becomes more intelligent and aware of the data traversing it. This allows you to secure critical business workloads in real-time right at the source of malicious traffic.
There is no configuration or additional costs to enable ThreatIQ on the Aviatrix Secure Networking Platform. Any Aviatrix customers with CoPilot can take advantage of ThreatIQ with ThreatGuard.
To get started, launch the Sandbox Starter Tool or deploy directly from AWS Marketplace. If you’d like to learn more and see what Aviatrix can do for your cloud networking, reach out and set up a demo.
.
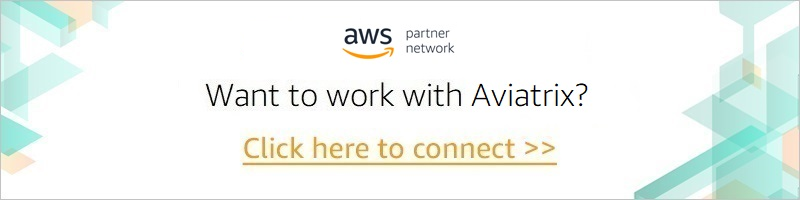
.
Aviatrix Systems – AWS Partner Spotlight
Aviatrix is an AWS Competency Partner that is uniquely positioned to provide deep insights into network traffic.
Contact Aviatrix | Partner Overview | AWS Marketplace
*Already worked with Aviatrix? Rate the Partner
*To review an AWS Partner, you must be a customer that has worked with them directly on a project.
Zero Friction AWS Lambda Instrumentation: A Practical Guide to Extensions
=======================
By Saar Tochner, Team Leader – Lumigo
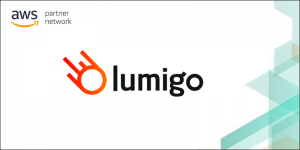 |
Lumigo |
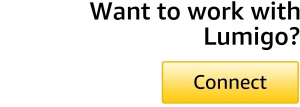 |
As serverless architectures start to grow, finding the right troubleshooting approach becomes a business-critical aspect.
In this post, I will dive into the “instrumentation approach” and how to keep track of internal events within an AWS Lambda function, and how to export processed telemetry data.
In my role at Lumigo, an AWS DevOps Competency Partner whose software-as-a-service (SaaS) platform helps companies monitor and troubleshoot serverless applications, I lead a team of extremely talented developers that builds the instrumentation tools of Lumigo.
The goal of my team is to provide frictionless instrumentation methods that users can instantly use in their serverless production environments. Here, I’ll share the knowledge on extensions that we gathered while pursuing these methods.
As with any real-life project, we will handle legacy code, multiple code owners, and a huge stack of serverless technologies. Our goal is to write as little code as possible, avoid changing existing code, support cross-runtime Lambda functions, and have no latency impact.
Below is a practical guide on how to use AWS Lambda Extensions. We’ll follow a storyline of extracting internal events from Lambda functions, processing them, and sending telemetry data to external services.
Big ambitions require great technology. Extensions to the rescue!
What are Troubleshooting Capabilities?
In a nutshell, a blindfolded programmer cannot achieve greatness solve bugs.
While working on serverless architectures, asynchronous operations happen all the time. A Lambda function triggered Amazon API Gateway, and an Amazon Simple Queue Service (SQS) message was written to Amazon Simple Storage Service (Amazon S3), while an Amazon DynamoDB stream triggers a Lambda function, and so on.
Troubleshooting a serverless architecture means being able to track down all of these events into a single “flow,” where the exception (when occurred) is on one end of the flow and the cause is on the other.
The main issue here is the data. We need to:
Collect every event which may be related or interesting.
Preprocess inside the Lambda environment (apply compliance rules, limit the size).
Export telemetry for further processing.
Considering a real-world approach, we should be able to do it everywhere:
Cross runtimes – be independent of the Lambda function’s runtime.
No latency impact – the Lambda function should respond as fast as before.
Bulletproof – never change the Lambda function’s flow.
We’ll use internal and external extensions to achieve our goals, as shown in this Lumigo blog post. Following, I’ll discuss two interesting features that are the core of our implementation: post-execution processing and pre-execution processing with wrappers.
Post-Execution Processing with External Extensions
External extensions are hooks the Amazon Web Services (AWS) infrastructure provides inside the Lambda container. The code of the extension runs independently of the Lambda runtime process and is integrated into the Lambda lifecycle.
By communicating with the Lambda function, the extension can gather information and process it in the post-execution time. This occurs after the Lambda function has returned its response and before the extensions are finished running.
More information about the lifecycle of a Lambda function can be found in the AWS documentation.
Being an external process allows us to write it in our favorite language, and it will work on any Lambda runtime (assuming the extension is wrapped as an executable binary).
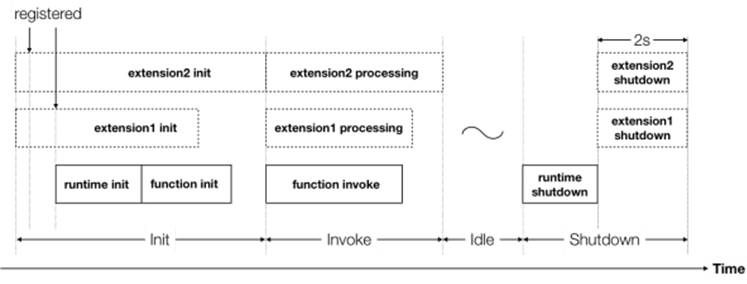
Figure 1 – AWS Lambda function lifecycle.
During the post-execution phase, we can process and export the telemetry data without interfering with the core invocation phase. Thus, we can avoid latency impact on the Lambda function, and the response will return as fast as it would without the extension.
At Lumigo, we use this phase to enforce privacy guarantees on the exported data (such as masking secrets and domains), and to ensure no private data escapes the Lambda environment. We use this timespan to limit data size and execute the exporting request itself.
Pre-Execution Processing with Wrappers
Wrappers, or internal extensions, are executable files that are executed during the container’s cold initialization, just before the runtime is initialized. It can be used to alter the runtime, modify environment variables, and execute code at the beginning of the process.
In our case, we use a wrapper to define the environment hooks that communicate with the external extension and transfer data. The external extension will later (in the post-execution time) take this data, process it, and export it.
This is a critical phase where we add some code lines that run in the Lambda function itself. More information about the communication methods between the internal and external extensions will be discussed in the next section.
At Lumigo, we’re wrapping all the HTTP requests of the Lambda function. When a Lambda function creates a request, we catch it and send it to the external extension. Using these sensors, we can reconstruct the full “flow” of the serverless architecture.
Extensions and Hooks Communication
To “move” the CPU or time-extensive logic from the execution phase to the post-execution phase, we must transfer the event’s data from the runtime’s process to the extension process.
There are different architectures that fit this use case and each has its own pros and cons.
Following, we’ll compare the most popular approaches: server-client, file system, and plain IPC.
In the performance tests, we transferred 1MB of data and checked it with Lambda function memory sizes: 128MB, 256MB, and 512MB.
- Server-client communication: The external extension serves as the server, and the hooks post data to it. An obvious pro here is that this is the most simple and elegant method. Another pro is reliability (the extension can return an acknowledgment, or ACK) which is achieved using TCP.
.
On the other hand, the performances are low: 80ms (128MB), 38ms (256MB), and 10ms (512MB). Slow or malfunctioned extensions may damage the execution time even more (due to the wait for an ACK).
.
- File-system communication: The hooks write data to a special directory, and the extension reads from it only during the post-execution time. The biggest pro here is the lack of coupling between the two processes. The Lambda function’s main process will never be affected if the extension malfunctions.
.
On the other hand, the API should be implemented with care (in order to avoid miscommunication that may cause timeouts) and there is no reliability. The performances are much better here, with: 5.56ms (128MB), 3ms (256MB), and 1ms (512MB).
.
- Intra-process-communication (IPC): The extensions and hooks communicate over operating system (OS) syscalls. This is a very raw method and, thus, very fast (2-3 microseconds), but it’s hard to implement and doesn’t have any out-of-the-box reliable communication.
To sum it up, there are many different architectures with their own pros and cons. Your specific use case should inform the choice of one over the other. A brief summary can be found in the following table:
|
Performance (128 / 256 /512MB) |
Reliability |
Internal-External Decoupling |
Implementation Size |
Server-Client |
80 / 38 /10ms |
Yes |
No |
Small |
File-System |
5.56 / 3 / 1ms |
No |
Yes |
Medium |
IPC |
~2.5 μs |
No (no out-of-the-box solution) |
No |
Large |
At Lumigo, we decided to use the file-system approach, as it’s relatively clean to implement and ensures high decoupling between the processes. This way, we answer to both performance and isolation requirements, and also write elegant code that can be maintained better.
Implementation
External Extension
An external extension is an executable file that contains two important parts: the register and the extension loop.
We decided to write our extension in a higher-level language (Node.js 14.x) and compiled it into an executable that could be run in the Lambda runtime environment.
In order for the Lambda service to identify this file as an extension, it needs to be executable and packed inside a layer, under a directory named ‘extensions’.
Register happens in the bootstrap time of the container and is the first communication with the Lambda service:
def register_extension() -> str:
body: str = json.dumps({"events": ["INVOKE", "SHUTDOWN"]})
headers: dict = {"Lambda-Extension-Name": "extension-name"}
conn = http.client.HTTPConnection(os.environ["AWS_LAMBDA_RUNTIME_API"])
conn.request("POST", "/2020-01-01/extension/register", body, headers=headers)
extension_id: str = conn.getresponse().headers["Lambda-Extension-Identifier"]
get_logger().debug(f"Extension registered with id {extension_id}")
return extension_id
Note that a common fallback here is the “extension-name” should be equal to the name of the file. More information can be found in the AWS documentation.
Next, the extension loop queries for the next event from the Lambda service:
def extension_loop(extension_id):
url = (
f"http://{os.environ['AWS_LAMBDA_RUNTIME_API']}/2020-01-01/extension/event/next"
)
req = urllib.request.Request(url, headers={HEADERS_ID_KEY: extension_id})
while True:
event = json.loads(urllib.request.urlopen(req).read())
get_logger().debug(f"Extension got event {event}")
handle(event)
if event.get("eventType") == "SHUTDOWN":
get_logger().debug(f"We're in the shutdown phase")
break
This ‘urlopen’ request blocks the execution of the program when there’s no waiting invocation.
Note there is no need to define a timeout here; the container is being halted when there is no invocation, so this call will not get enough CPU to reach its timeout.
More in-depth code examples can be found in AWS official samples.
Internal Extension
In general, a do-nothing internal extension is much simpler and looks like this:
#!/bin/bash
exec "$@"
In order for the Lambda service to use this wrapper script, we need to add the environment variable AWS_LAMBDA_EXEC_WRAPPER
with the path to this file.
At Lumigo, we use different methods to wrap the HTTP requests in different runtimes. For example, in the pythonic wrapper, we use the library wrapt to keep track of the most commonly-used function for HTTP communication (used by AWS SDK, requests, and many others):
wrap_function_wrapper("http.client", "HTTPConnection.__init__", _http_init_wrapper)
Conclusion
Using the advanced tools that AWS Lambda provides, you can create robust mechanisms that answer core demands in serverless architectures.
In this post, I explored how to use external and internal extensions to create an instrumentation tool that extracts data from the Lambda function, processes, and exports it, and with almost no latency hit.
I also showed how most of the code could be written just once, in the external extension part, thus avoiding unnecessary code repetition and allowing fast adoption across different runtimes in the project.
Check out Lumigo’s serverless solution to learn more. We already implemented all of the above, and more, to provide you with a full monitoring and troubleshooting solution, tailored to fit your serverless architectures.
The content and opinions in this blog are those of the third-party author and AWS is not responsible for the content or accuracy of this post.
.
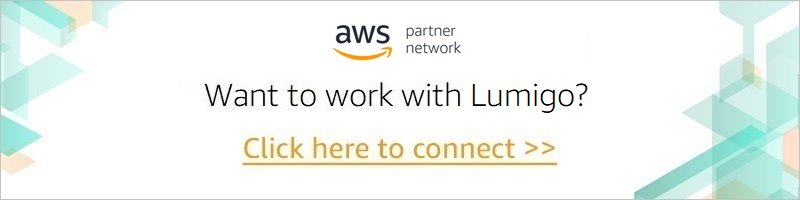
.
Lumigo – AWS Partner Spotlight
Lumigo is an AWS Competency Partner whose SaaS platform helps companies monitor and troubleshoot serverless applications.
Contact Lumigo | Partner Overview | AWS Marketplace
*Already worked with Lumigo? Rate the Partner
*To review an AWS Partner, you must be a customer that has worked with them directly on a project.
How to Build a Fintech App on AWS Using the Plaid API
=======================
By Rana Dutt, Sr. Solutions Architect – AWS
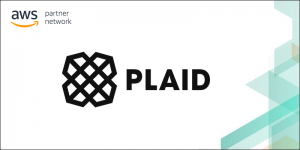 |
Plaid |
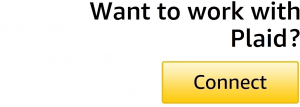 |
Open Finance initiatives have been gaining momentum across the world. These initiatives require that banks provide access to customer data through a common, open API for third-party applications, which are referred to as fintech apps.
The fintech app providers are generally not banks, but they offer users a variety of convenient payment and finance features on smartphone apps. These apps enhance the customer experience and foster greater choice and innovation. Users simply need to link the app to their bank and brokerage accounts, and grant the necessary permissions.
Fintech apps offer users benefits such as:
Viewing balances across multiple bank accounts.
Initiating payments to friends.
Applying for loans without gathering and scanning bank and income statements.
Paying for things online using a “Buy Now Pay Later” plan.
Showing monthly income and expense categories to help set budgets.
Displaying overall investment performance across multiple brokerage accounts.
Buying crypto-assets.
In this post, I will show you how to build and deploy a basic fintech app on Amazon Web Services (AWS) in under an hour by using the Plaid Link API. This app allows users to sign up, log in, select their bank from a list, connect to that bank, and display the latest transactions.
About Plaid
Plaid is a San Francisco-based financial services company and AWS Partner that helps fintech providers connect users safely to their bank accounts.
The Plaid Link acts as a secure proxy between a fintech app and a bank. With Plaid, application developers no longer need to worry about implementing scores of different ways to access data in myriad financial institutions.
Plaid is currently able to connect to more than 10,000 banks and financial institutions throughout the world. It provides a single API to connect to them. Currently, about 3,000 fintech apps use Plaid’s API to enable their users to access their bank accounts.
What We Will Build in This Post
Through this post, we will build a demo fintech app on AWS using the AWS Amplify framework and Plaid Link. AWS Amplify helps us quickly build a serverless web app with a React frontend, user sign-up and sign-in using Amazon Cognito, an Amazon API Gateway-based REST API, and an Amazon DynamoDB database for storage.
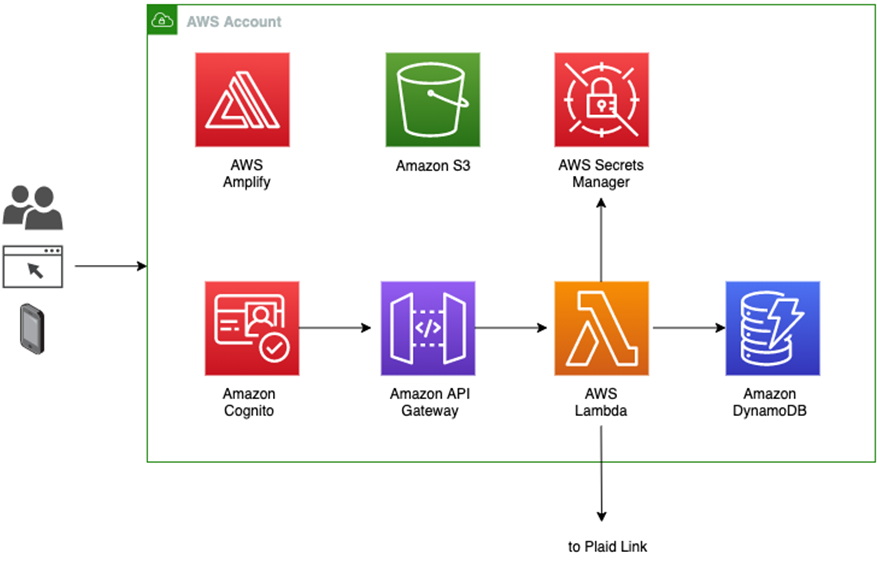
Figure 1 – Architecture of demo fintech app.
AWS Amplify generates the code for signing up and authenticating users who are then stored in a Cognito user pool. It also helps create a REST API invoked by the React frontend and implemented by an AWS Lambda function behind Amazon API Gateway. The backend Lambda function sets up the Plaid Link which allows the end user to interact with a selected bank.
AWS Amplify also helps store the Plaid API key securely in AWS Secrets Manager so that it never needs to appear in the code or in a file. Plaid access tokens (described in the next section) are stored in the DynamoDB database.
This is a completely scalable and secure architecture which does not require the user to manage any server instances.
How Plaid Link Works
To build an app using Plaid Link, you first need to go to Plaid.com, click on the Get API Keys button, and create an account. You can create a free sandbox account to start.
You can then log into your dashboard and find your sandbox API key under the menu for Team Settings – Keys.
The following diagram shows what our demo Web app needs to implement.
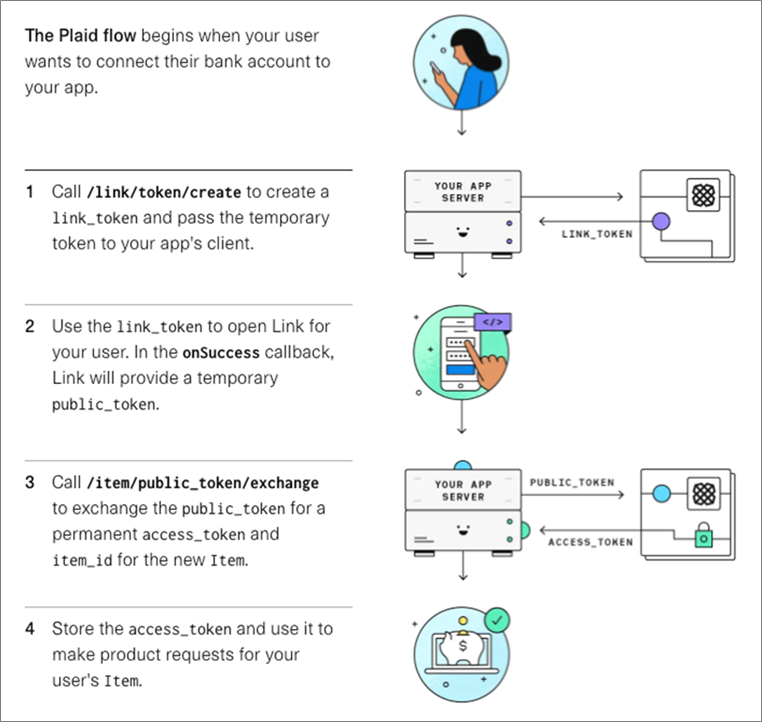
Figure 2 – Plaid Link flow.
All API calls are made through a Plaid client object. The message flow is as follows:
- The app first creates a Plaid client object by passing in the Plaid API key and Plaid client ID. It then calls the client’s createLinkToken method to obtain a temporary link token.
- When the user selects a bank, the app uses the link token to open a Plaid Link to the bank and obtain a temporary public token.
- The app then calls the client object’s exchangePublicToken method to exchange the public token for a permanent access token and an item ID that represents the bank.
- The app stores the access token in DynamoDB for subsequent requests pertaining to that item. For example, the app can pass the access token to the client object’s getTransactions method to obtain a list of transactions within a specific date range.
Building and Deploying the App
Prerequisites
Make sure you have created a sandbox account at Plaid as described above, and obtained your API keys.
You also need to install AWS Amplify.
If you have not already done so, create a default AWS configuration profile by running the aws configure command.
Building the App
Clone the repo and run npm install:
$ git clone https://github.com/aws-samples/aws-plaid-demo-app.git
$ cd aws-plaid-demo-app
$ npm install
Initialize a new Amplify project. Hit Return to accept the defaults.
$ amplify init
? Enter a name for the project (awsplaiddemoapp)
? Initialize the project with the above configuration? (Y/n) y
? Select the authentication profile you want to use: (Use arrow keys)
> AWS profile
? Please choose the profile you want to use: (Use arrow keys)
> default
…
Your project has been successfully initialized and connected to the cloud!
Add authentication:
$ amplify add auth
? Do you want to use the default authentication configuration?
> Default configuration
? How do you want users to be able to sign in? (Use arrow keys and space bar to select)
• Email
• Username
? Do you want to configure advanced settings?
> No, I am done
Add the API:
$ amplify add api
? Please select from one of the below mentioned services: REST
? Provide a friendly name for your resource to be used as a label for this category in the project: plaidtestapi
? Provide a path (e.g., /book/{isbn}): /v1
? Choose a Lambda source: Create a new Lambda function
? Provide an AWS Lambda function name: plaidaws
? Choose the runtime that you want to use: NodeJS
? Choose the function template that you want to use: Serverless ExpressJS function (Integration with API Gateway)
? Do you want to configure advanced settings? Yes
? Do you want to access other resources in this project from your Lambda function? No
? Do you want to invoke this function on a recurring schedule? No
? Do you want to enable Lambda layers for this function? No
? Do you want to configure environment variables for this function? Yes
? Enter the environment variable name: CLIENT_ID
? Enter the environment variable value: [Enter your Plaid client ID]
? Select what you want to do with environment variables: Add new environment variable
? Select the environment variable name: TABLE_NAME
? Enter the environment variable value: plaidawsdb
? Select what you want to do with environment variables: I am done
? Do you want to configure secret values this function can access? Yes
? Enter a secret name (this is the key used to look up the secret value): PLAID_SECRET
? Enter the value for PLAID_SECRET: [Enter your Plaid sandbox API key - hidden]
? What do you want to do? I'm done
? Do you want to edit the local lambda function now? No
? Restrict API access: No
? Do you want to add another path? No
Copy the Lambda source file, install dependencies, and push:
$ cp lambda/plaidaws/app.js amplify/backend/function/plaidaws/src/app.js
$ cd amplify/backend/function/plaidaws/src
$ npm i aws-sdk moment plaid@8.5.4
$ amplify push
Add a database:
$ amplify add storage
? Please select from one of the below mentioned services: NoSQL Database
? Please provide a friendly name for your resource that will be used to label this category in the project: plaidtestdb
? Please provide table name: plaidawsdb
You can now add columns to the table.
? What would you like to name this column: id
? Please choose the data type: string
? Would you like to add another column? Yes
? What would you like to name this column: token
? Please choose the data type: string
? Would you like to add another column? No
? Please choose partition key for the table: id
? Do you want to add a sort key to your table? No
? Do you want to add global secondary indexes to your table? No
? Do you want to add a Lambda Trigger for your Table? No
Successfully added resource plaidtestdb locally
Update the Lambda function to add permissions for the database:
$ amplify update function
? Select the Lambda function you want to update plaidaws
General information
- Name: plaidaws
- Runtime: nodejs
Resource access permission
- Not configured
Scheduled recurring invocation
- Not configured
Lambda layers
- Not configured
Environment variables:
- CLIENT_ID: plaidclientid
Secrets configuration
- PLAID_SECRET
? Which setting do you want to update? Resource access permissions
? Select the categories you want this function to have access to.
storage
? Storage has 2 resources in this project. Select the one you would like your Lambda to access plaidawsdb
? Select the operations you want to permit on plaidawsdb: create, read, update, delete
? Do you want to edit the local lambda function now? No
Deploying the App
Add hosting for the app:
$ amplify add hosting
? Select the plugin module to execute:
> Hosting with Amplify Console (Managed hosting)
? Choose a type
> Manual deployment
Deploy the app:
$ amplify publish
Testing the App
Go to the URL displayed by the amplify publish command, and sign up as a new user. After logging in, select a bank from the list displayed.
If you are using the sandbox environment, use the credentials user_good / pass_good to access the bank and display the transactions.
Conclusion
The walkthrough in this post demonstrates how easy it is to use AWS Amplify to create a secure, scalable, and completely serverless fintech app on AWS that allows users to sign up, select from among the 10,000 banks that Plaid Link connects to, and obtain the transaction history for a particular account.
From here, you can add features such as making payments to friends or vendors, displaying balances across multiple accounts, sending low balance alerts and helping set a budget.
.
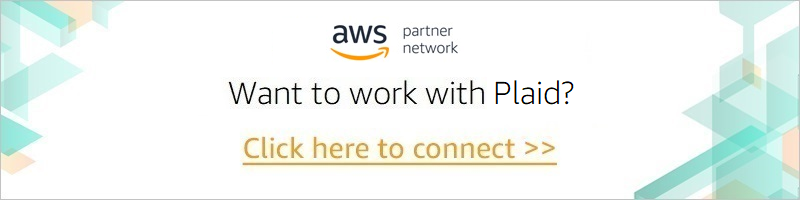
.
Plaid – AWS Partner Spotlight
Plaid is an AWS Partner that helps fintech providers connect users safely to their bank accounts.
Contact Plaid | Partner Overview
*Already worked with Plaid? Rate the Partner
*To review an AWS Partner, you must be a customer that has worked with them directly on a project.
Accelerate Your SAP S/4HANA Migration to AWS and Utilize Nearly 50 Years of IBM’s SAP Experience
=======================
By Naeem Asghar, Sr. Partner Solution Architect – AWS
By Vamsi Yanamadala, Sr. Partner Solution Architect – AWS
By Zoe Tomkins, Principle Partner Development Manager – AWS
By Jasbir Singh, IBM Global Lead, Accelerated Move Centre (AMC) – IBM
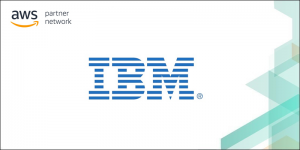 |
IBM |
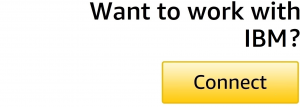 |
This post explains the challenges faced by customers running SAP ECC environments on premises and how IBM can accelerate their S/4 HANA transformation journey to Amazon Web Services (AWS).
IBM Accelerated Move Centre (AMC) delivers a cost-effective approach to SAP S/4HANA transformations:
Migrate and modernize at your own pace, adopting new technologies with accelerators that meet the specific requirements of your organization.
Utilize existing investments by preserving proven business processes and data models.
Reduce costs and time-to-ROI by minimizing upfront investments in new infrastructure.
Embrace cloud innovations by quickly leveraging new AWS technologies with minimal change to your existing business.
IBM is an AWS Premier Consulting Partner with 10 AWS Competencies including the AWS SAP Competency and 13 AWS service validations.
IBM also participates in AWS Partner programs such as AWS Migration Acceleration Program (MAP), which offsets the migration costs of cloud adoption for customers and accelerates migration with additional AWS resources.
Customer Success Story
Customers face challenges when migrating and modernizing legacy SAP systems, and that was the case for a large process manufacturing company based in India which was experiencing issues with old hardware due for a refresh.
This included 13 years of legacy data, old versions of Oracle DB, complex interfaces and customer objects, performance issues with ABAP objects, material requirement planning, and Batch Management functionality for finished goods that was not performant.
The IBM Accelerated Move Centre (AMC) solution is a brownfield transformation to SAP S/4 HANA in a single step. By utilizing this solution, IBM was able to migrate the customer to SAP S/4HANA quickly and efficiently, while also upgrading Solution Manager and activating Batch Management to monitor and track finished goods.
The customer saw 100% capital cost avoidance on new on-premises infrastructure, 30% faster application response times for business users, shorter approval duration to due to workflow implementation, overall data footprint reduction, and an overall reduction in storage costs.
The organization was also able to automate 85% of their data conversion processes during the SAP transformation. To learn more, read the full case study.
The Solution
IBM and AWS have the experience and knowledge to streamline complex SAP migrations and enable organizations to fully leverage the breadth of AWS tools and services that modernize SAP workloads.
AWS is the most reliable cloud to run your SAP workloads, providing the longest track record of cloud success and supporting more than 5,000 active SAP customers. Get the assistance you need to execute migrations, undergo transformation, or improve how you run SAP by leveraging IBM and their proven expertise.
With AWS, businesses can confidently run SAP systems with SAP-certified infrastructure that can support even the most demanding HANA-based production deployments.
Together, IBM and AWS offer a practical and cost-effective process for transformations to SAP S/4HANA, pre-scoped to fit your business requirements and drive future adoption of innovative SAP solutions.
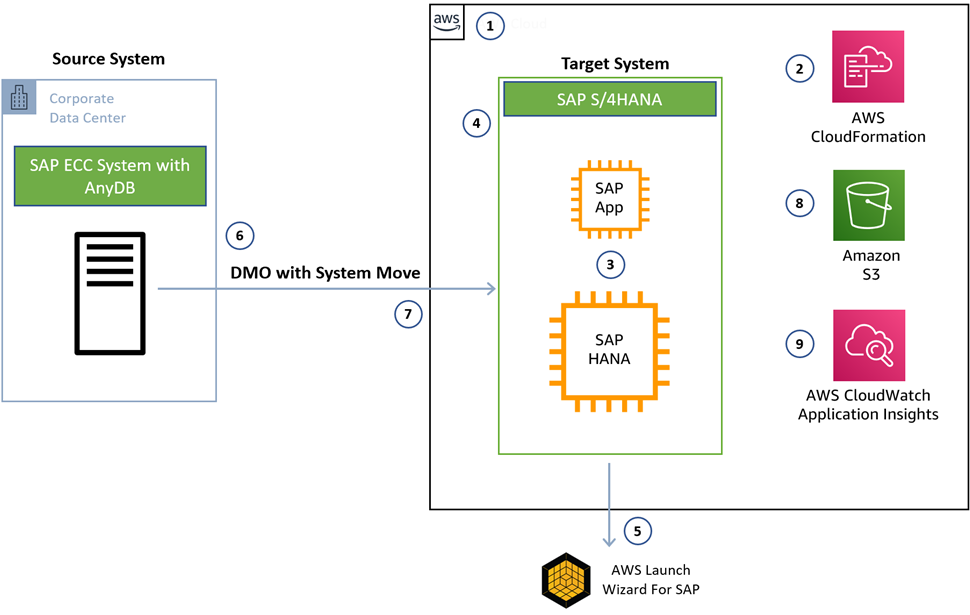
Figure 1 – Accelerated S/4 HANA conversion with AWS services.
- Design the AWS landing zone based on security and compliance needs of the customer.
- Deploy the landing zone as infrastructure as code (IaC) with AWS CloudFormation.
- Size out SAP system based on SAP HANA Sizing and business needs.
- Understand and design high availability and disaster recovery (DR) requirements based recovery point objective (RPO) and recovery time objective (RTO) requirements.
- Utilize the AWS Launch Wizard for SAP to deploy the SAP S/4 HANA target system in just over two hours.
- Start Software Update Manager (SUM) with the system update and database migration process.
- Finish the system update and database migration procedure on the target system.
- Install and configure the AWS Backint Agent to manage and save SAP HANA backups to Amazon Simple Storage Service (Amazon S3). AWS Backint Agent is SAP-certified and integrates with SAP management tools.
- Configure the SAP HANA database with Amazon CloudWatch Application Insights to monitor the health of your of the HANA database.
IBM AMC is a next-generation factory migration solution designed to migrate and modernize SAP landscapes to SAP S/4HANA on AWS with pre-scoped solutions to fit the specific requirements of each business and its SAP S/4HANA transformation goals.
Keeping in line with customer requirements and market trends, the IBM AMC solution is pre-scoped into T-shirt sizes to accommodate all types of customers. Each T-shirt size comes with a pre-defined system architecture, delivery model, and project plan.
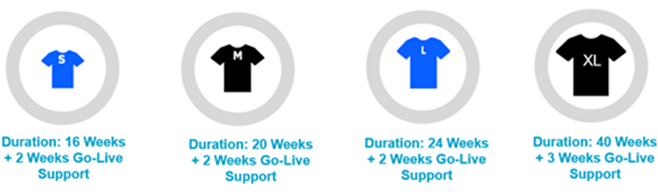
Figure 2 – Pre-scoped T-shirt sizes.
Regardless of the T-shirt size, all IBM AMC projects are scoped with basis and security, development, functional, and testing capabilities.
IBM AMC has developed key differentiators with their tooling and accelerators, including:
IBM’s HANAtization console that identifies custom objects for code remediation and saves up to 60% of manual effort.
IBM Finance Reconciliation tool which allows the execution of pre- and post-reports for general ledger, vendor, customer, and cost center including assets.
IBM Stock Reconciliation tool which assists in stock reconciliation after the SAP HANA conversion and covers all types of stock locations, including both managed and split valuated stock.
These tools have been assessed by SAP and declared as market differentiators and help reduce project timelines and costs, while ensuring business continuity.
The value proposition to customers is quantifiable. With benchmarks based on different projects starting from the preparation phase, actual execution of the project, and then moving to the new system.
Some of the benefits realized are:
Automation for code remediation to reduce project timelines.
Downtime reduction using reconciliation tools.
Reduction of preparatory work and time through tools in finance and master data.
Reduction of time in managed fixes and code changes, reducing effort needed by maintenance teams.
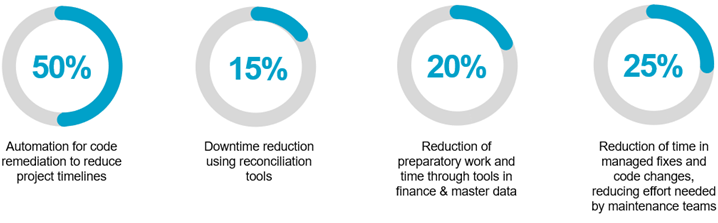
Figure 3 – IBM tooling value proposition.
The IBM AMC offering also supports RISE with SAP, a business transformation as a service to help clients shift to the intelligent enterprise.
SAP Digital Transformation with IBM
IBM AMC is a central part of the strategic evolution partnership between IBM and SAP. As a global leader in SAP transformations, IBM offers in-depth industry and process experience to make your business smarter.
Together with SAP, IBM and AWS can help make your digital transformation a reality, bringing intelligence to your entire enterprise.
IBM’s benefits include:
Industry innovation focused on integrated and intelligent processes leveraging IBM and SAP industry best practices to reimagine operational excellence and drive new business value.
Experience management to reimagine customer and employee experiences by capitalizing on built-in industry intelligent workflows.
Data transformation using SAP solutions to help you transform data into trusted business value and a reference architecture to build intelligent cloud solutions.
Roadmap development for private deployments of SAP Cloud Platform with IBM Red Hat OpenShift.
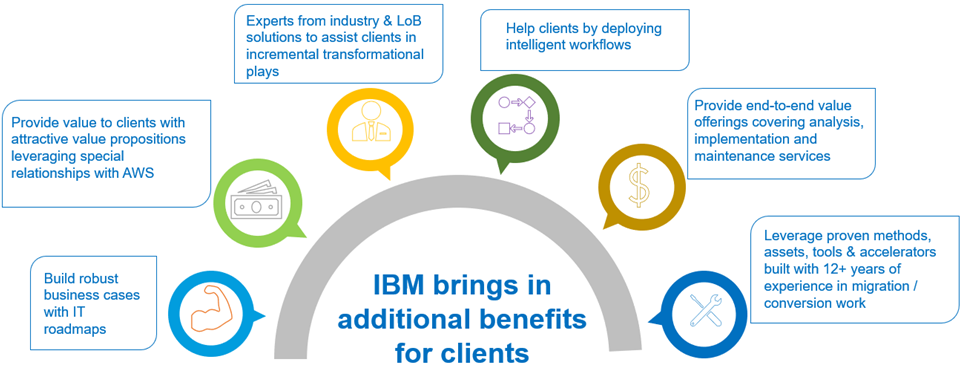
Figure 4 – IBM’s experience and benefits.
Post deployment, there are several AWS services available to help automate DevOps-based management for SAP.
IBM delivers this automation for infrastructure provisioning, SAP installation including SAP clusters by leveraging AWS Launch Wizard, auto scaling, SAP kernel patch automation, HANA upgrades using AWS Systems Manager, and monitoring SAP systems via Amazon CloudWatch.
To learn more, see the Get Started with SAP on AWS page.
Conclusion
IBM provides the experience, tooling, methodology, and best practices to streamline your SAP transformation, combining SAP S/4HANA conversion and migration to AWS in a single step using the IBM’s Accelerated Move Centre (AMC).
IBM has nearly 50 years of experience in the industry, where no other SAP partner has more Global Partner Program Certifications. IBM has also completed over 6,500 SAP projects including hundreds of SAP S/4HANA implementations.
.
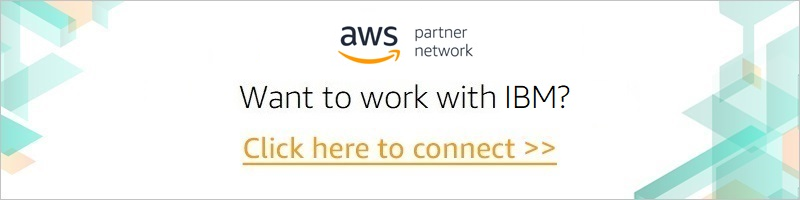
.
IBM – AWS Partner Spotlight
IBM is an AWS Premier Consulting Partner and MSP that offers comprehensive service capabilities addressing both business and technology challenges that clients face today.
Contact IBM | Partner Overview
*Already worked with IBM? Rate the Partner
*To review an AWS Partner, you must be a customer that has worked with them directly on a project.
Building a Multi-Region Solution for Auto Recovery of Amazon EC2 Instances Using AWS CDK and AWS Step Functions
=======================
By Rafal Krol, Cloud SRE – Chaos Gears
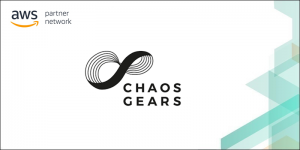 |
Chaos Gears |
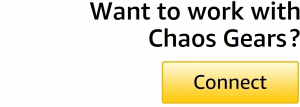 |
At Chaos Gears, an AWS Advanced Consulting Partner, we help customers and companies of all sizes utilize Amazon Web Services (AWS) to its full potential so they can focus on evolving their business.
One of our customers, a startup from the medical industry, has gained a global reach and serves its clients by operating in multiple AWS regions (currently 10 with more scheduled to come) spanning many time zones.
At the center of each region, there’s an Amazon Elastic Compute Cloud (Amazon EC2) instance that, by design, occasionally maxes out on the CPU. When this happens, a member of the customer’s operations team runs a set of checks to determine whether the instance is reachable; if it’s not, it gets restarted.
Once restarted and back online, which takes a few minutes, the same round of checks recommences. More often than not, this approach proves sufficient and the on-call engineer handling the issue can get back to work.
Being a startup and lacking the resources to man a follow-the-sun operations team, the customer came to Chaos Gears requesting a simple, adjustable, and cost-effective solution that would relieve their engineers from such an operational burden.
This post looks at the multi-regional first-line of support solution Chaos Gears built for the customer. I will also discuss how we automated the incident response duties that would typically engage at least one of the first-line of support engineers.
Before launching this solution, the on-call engineers needed to identify the affected instances, spread among different AWS regions, manually run a set of pre-defined checks on each one, and, based on the outcome, either do nothing or restart the pertinent machines and rerun the checks.
Infrastructure as Code
In today’s world of agile software development, our team at Chaos Gears treats everything as code, or at least we should be doing that.
Hence, the first decision we made for the customer was to leverage the AWS Cloud Development Kit (AWS CDK), a multi-language software development framework for modelling cloud infrastructure as reusable components as our infrastructure as code (IaC) tool.
Our customer’s software engineers were already familiar with TypeScript, the language we chose to build out the infrastructure with, which meant they’d comprehend the final solution quickly.
Moreover, we avoided the steep learning curve of mastering a domain-specific language (DSL) and the additional burden of handling an unfamiliar codebase.
The recent introduction of ASW CDK integration with the AWS Serverless Application Model (SAM), allows for developing serverless applications seamlessly within a CDK project.
On top of all of that, we could reuse the existing software tooling like linters and apply the industry’s coding best practices.
Serverless
The adage says that “no server is better than no server,” and with that in mind we turned our heads towards AWS Step Functions, a serverless orchestrator for AWS Lambda and other AWS services.
The challenge at hand was perfect for an event-driven architecture, and we had already envisioned the subsequent steps of the verification process:
URL health check
Amazon Route 53 health check
SSH check
Restart
We needed the glue, and with AWS Step Functions we effortlessly combined all of those pieces without worrying about server provisioning, maintenance, retries, and error handling.
Managed Services
We had the backbone figured out, but we still had to decide how to monitor the CPU usage on the Amazon EC2 instances and pass the knowledge of a breach to AWS Step Functions state machine.
It screamed of Amazon CloudWatch alarms for the metric monitoring bit and Amazon EventBridge for creating a rule for routing the alarm event to the target (a state machine, in our case).
Business Logic
When the ‘CPUUtilization’ metric for a given instance reaches 100%, a CloudWatch alarm enters the ‘alarm’ state. This change gets picked up by an EventBridge rule that triggers the AWS Step Functions state machine.
Upon receiving the event object from the EventBridge rule, the state machine orchestrates the following workflow:
- Three checks run, one after another—URL check, Route 53 check, and SSH check.
- If all checks succeed during the first run, the execution ends silently (the ‘All good’ step followed by the ‘End’ field).
- When a check fails, the EC2 instance is restarted and we recommence from the beginning with a second run.
- If all checks succeed during the second run, a Slack notification is sent and the execution ends (the ‘Slack’ step followed by the ‘End’ field).
- When a check fails during the second run, an OpsGenie alert is created and the execution ends (the ‘OpsGenie’ step followed by the ‘End’ field).
Here’s the diagram depicting the complete solution:
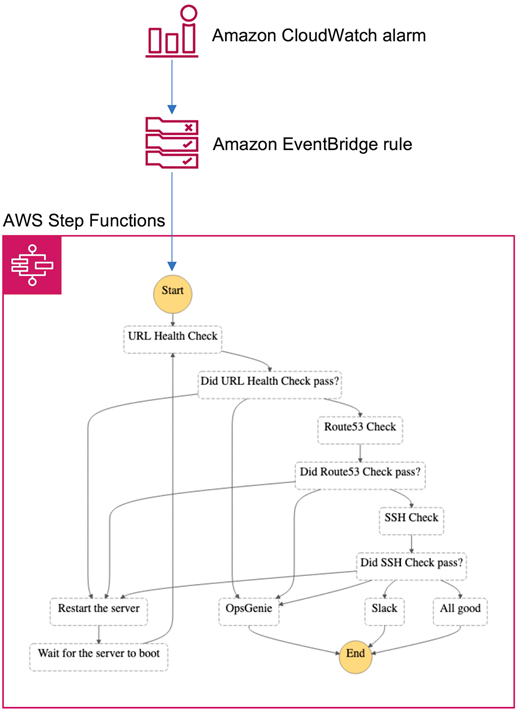
Figure 1 – State machine.
All of the above-mentioned resources, plus the Lambda functions, an Amazon Simple Storage Service (Amazon S3) bucket for the Lambda code packages, and the necessary AWS Identity and Access Management (IAM) roles and policies are created and managed by AWS CDK and AWS SAM.
Furthermore, this solution can be deployed effortlessly to multiple regions using AWS CDK environments.
A Peek at the Code
A public repository is available on GitHub with a full working solution and a detailed README. I won’t dissect all of the code here, but let me draw your attention to some of the more interesting elements.
In the project’s root directory, we keep a ‘tsconfig.json’ file responsible for configuring the TypeScript’s compiler, and an ‘.eslintrc.json’ file holding the configuration for ES Lint, a popular JavaScript linter.
These two configuration files serve the entire project since we use TypeScript for both the infrastructure and application layers.
AWS CDK’s support for many popular general-purpose languages (TypeScript, JavaScript, Python, Java, C#, and Go, which is in developer preview) enables and encourages the DevOps culture by making the end-to-end development experience more uniform, as you can use familiar tools and frameworks across your whole stack.
Now, let’s take a closer look at the ‘bin/cpu-check-cdk.ts’ file, the point of entry to our CDK app, whence all stacks are instantiated.
We imported all of the necessary dependencies one library at a time, but in AWS CDK v2 all of the CDK libraries are consolidated in one package.
```typescript
#!/usr/bin/env node
import 'source-map-support/register'
import * as cdk from '@aws-cdk/core'
import * as iam from '@aws-cdk/aws-iam'
```
Next, we check whether all of the necessary environment variables have been set.
```typescript
import { SLACK_TOKEN, SLACK_CHANNEL_ID, TEAM_ID, API_KEY, R53_HEALTH_CHECK_ID } from '../config'
if (!SLACK_TOKEN) {
throw new Error('SLACK_TOKEN must be set!')
}
if (!SLACK_CHANNEL_ID) {
throw new Error('SLACK_CHANNEL_ID must be set!')
}
if (!TEAM_ID) {
throw new Error('TEAM_ID must be set!')
}
if (!API_KEY) {
throw new Error('API_KEY must be set!')
}
if (!R53_HEALTH_CHECK_ID) {
throw new Error('R53_HEALTH_CHECK_ID must be set!')
}
```
Then, we initialize the CDK app construct.
```typescript
const app = new cdk.App()
```
We grab the regions to which to deploy, along with corresponding instance IDs to monitor from AWS CDK’s context.
```typescript
const regionInstanceMap: Map<string, string> = app.node.tryGetContext('regionInstanceMap')
```
Next, we create a tags object with the app’s version and repo’s URL taken directly from the package.json file.
```typescript
import { repository, version } from '../package.json'
const tags = {
version,
repositoryUrl: repository.url,
}
```
Finally, we loop through the map of regions and corresponding instance IDs we grabbed earlier.
In each region, we produce eight stacks: one for every Lambda function, one for the state machine, and one for the metric, alarm, and rule combo.
```typescript
import { StateMachineStack } from '../lib/state-machine-stack'
import { LambdaStack } from '../lib/lambda-stack'
import { MetricAlarmRuleStack } from '../lib/metric-alarm-rule-stack'
for (const [region, instanceId] of Object.entries(regionInstanceMap)) {
const env = {
region,
account: process.env.CDK_DEFAULT_ACCOUNT,
}
const lambdaStackUrlHealthCheck = new LambdaStack(app, `LambdaStackUrlHealthCheck-${region}`, {
tags,
env,
name: 'url-health-check',
policyStatementProps: {
effect: iam.Effect.ALLOW,
resources: ['*'],
actions: ['ec2:DescribeInstances'],
},
})
const lambdaStackR53Check = new LambdaStack(app, `LambdaStackR53Check-${region}`, {
tags,
env,
name: 'r53-check',
policyStatementProps: {
effect: iam.Effect.ALLOW,
resources: [`arn:aws:route53:::healthcheck/${R53_HEALTH_CHECK_ID}`],
actions: ['route53:GetHealthCheckStatus'],
},
environment: {
R53_HEALTH_CHECK_ID,
},
})
const lambdaStackSshCheck = new LambdaStack(app, `LambdaStackSshCheck-${region}`, {
tags,
env,
name: 'ssh-check',
policyStatementProps: {
effect: iam.Effect.ALLOW,
resources: ['*'],
actions: ['ec2:DescribeInstances'],
},
})
const lambdaStackRestartServer = new LambdaStack(app, `LambdaStackRestartServer-${region}`, {
tags,
env,
name: 'restart-server',
policyStatementProps: {
effect: iam.Effect.ALLOW,
resources: [`arn:aws:ec2:${region}:${process.env.CDK_DEFAULT_ACCOUNT}:instance/${instanceId}`],
actions: ['ec2:RebootInstances'],
},
})
const lambdaStackSlackNotification = new LambdaStack(app, `LambdaStackSlackNotification-${region}`, {
tags,
env,
name: 'slack-notification',
environment: {
SLACK_TOKEN,
SLACK_CHANNEL_ID,
},
})
const lambdaStackOpsGenieNotification = new LambdaStack(app, `LambdaStackOpsGenieNotification-${region}`, {
tags,
env,
name: 'opsgenie-notification',
environment: {
TEAM_ID,
API_KEY,
EU: 'true',
},
})
const stateMachineStack = new StateMachineStack(app, `StateMachineStack-${region}`, {
tags,
env,
urlHealthCheck: lambdaStackUrlHealthCheck.lambdaFunction,
r53Check: lambdaStackR53Check.lambdaFunction,
sshCheck: lambdaStackSshCheck.lambdaFunction,
restartServer: lambdaStackRestartServer.lambdaFunction,
slackNotification: lambdaStackSlackNotification.lambdaFunction,
opsGenieNotification: lambdaStackOpsGenieNotification.lambdaFunction,
})
new MetricAlarmRuleStack(app, `MetricAlarmRuleStack-${region}`, {
tags,
env,
instanceId,
stateMachine: stateMachineStack.stateMachine,
})
}
```
Thanks to using a basic programming concept of the for loop, we saved ourselves from unnecessary duplication by keeping things DRY (Don’t Repeat Yourself).
Nice and easy, and all in one go, regardless of the number of regions to which we would want to deploy, and mind you, there are 25 available (with more to come).
I won’t be going through all of the AWS CDK and Lambda files in this post, though I strongly encourage you to give the code a thorough review.
Notwithstanding, let’s see how easy it is to define a stack class in AWS CDK looking at the `lib/lambda-stack.ts` file. First, we import the dependencies:
```typescript
import * as cdk from '@aws-cdk/core'
import * as iam from '@aws-cdk/aws-iam'
import * as lambda from '@aws-cdk/aws-lambda'
import { capitalizeAndRemoveDashes } from './helpers'
```
You’ll notice there is a helper function called `capitalizeAndRemoveDashes` amongst the CDK libs. Since AWS CDK uses a GPL, we can introduce any amount of custom logic, as we could do with a _regular_ application.
The `lib/helpers.ts` file looks as follows:
```typescript
/**
* Take a kebab-case string and turn it into a PascalCase string, e.g.: my-cool-function -> MyCoolFunction
*
* @param kebab
* @returns string
*/
export function capitalizeAndRemoveDashes(kebab: string): string {
const kebabSplit = kebab.split('-')
for (const i in kebabSplit) {
kebabSplit[i] = kebabSplit[i].charAt(0).toUpperCase() + kebabSplit[i].slice(1)
}
return kebabSplit.join('')
}
```
Next, we extend the default stack properties like description with ours, setting some as mandatory and some as optional.
```typescript
interface LambdaStackProps extends cdk.StackProps {
name: string,
runtime?: lambda.Runtime,
handler?: string,
timeout?: cdk.Duration,
pathToFunction?: string,
policyStatementProps?: iam.PolicyStatementProps,
environment?: {
[key: string]: string
},
}
```
We start a declaration of the `LambdaStack` class with a `lambdaFunction` read-only property and a constructor.
```typescript
export class LambdaStack extends cdk.Stack {
readonly lambdaFunction: lambda.Function
constructor(scope: cdk.Construct, id: string, props: LambdaStackProps) {
super(scope, id, props)
```
We then create a resource name out of the mandatory name property that will be passed in during the class initialization.
```typescript
const resourceName = capitalizeAndRemoveDashes(props.name)
```
We create an IAM role that the Lambda service can assume. We add the `service-role/AWSLambdaBasicExecutionRole` AWS-managed policy to it and, if provided, a custom user-managed policy.
```typescript
const role = new iam.Role(this, `Role${resourceName}`, { assumedBy: new iam.ServicePrincipal('lambda.amazonaws.com') })
role.addManagedPolicy(iam.ManagedPolicy.fromAwsManagedPolicyName('service-role/AWSLambdaBasicExecutionRole'))
if (props.policyStatementProps) {
role.addToPolicy(new iam.PolicyStatement(props.policyStatementProps))
}
```
Next, we initialize a construct of the Lambda function using the role defined earlier and stack properties, or arbitrary defaults if stack properties were not provided.
```typescript
const lambdaFunction = new lambda.Function(this, `LambdaFunction${resourceName}`, {
role,
runtime: props.runtime || lambda.Runtime.NODEJS_12_X,
handler: props.handler || 'app.handler',
timeout: props.timeout || cdk.Duration.seconds(10),
code: lambda.Code.fromAsset(`${props.pathToFunction || 'src'}/${props.name}`),
environment: props.environment,
})
```
Finally, we expose the Lambda function object as the class’s read-only property we defined earlier. We’re also sure to close our brackets to avoid the implosion of the universe.
```typescript
this.lambdaFunction = lambdaFunction
}
}
```
Conclusion
In this post, I showed how our team at Chaos Gears put together a serverless application running AWS Lambda under the baton of AWS Step Functions to relieve our customer’s engineers from some of their operational burdens. This enables them to focus more on evolving their business.
The approach described here can be adapted to serve other needs or cover different cases, as AWS Step Functions’ visual workflows allow for a quick translation of business requirements to technical ones.
By using AWS CDK as the infrastructure as code (IaC) tool, we were able to write all of the code in TypeScript, which puts us in an excellent position for future improvements.
We avoided the trap of introducing unnecessary complexity and kept things concise with codebase that was approachable and comprehensive to all team members.
Check out the GitHub repository and visit the Chaos Gears website to learn more about collaborating with us.
The content and opinions in this blog are those of the third-party author and AWS is not responsible for the content or accuracy of this post.
.
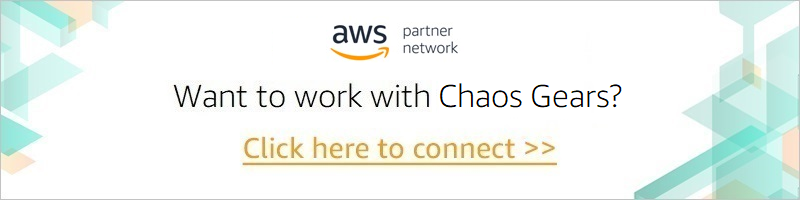
.
Chaos Gears – AWS Partner Spotlight
Chaos Gears is an AWS Partner that helps customers and companies of all sizes utilize AWS to its full potential so they can focus on evolving their business.
Contact Chaos Gears | Partner Overview
*Already worked with Chaos Gears? Rate the Partner
*To review an AWS Partner, you must be a customer that has worked with them directly on a project.
Building an Agile Business Rules Engine on AWS
=======================
By Kalyan Purkayashta, Sr. Manager – Capgemini
By Rakesh Porwad, Manager – Capgemini
By Vikas Nambiar, Manager, Partner Solutions Architect – AWS
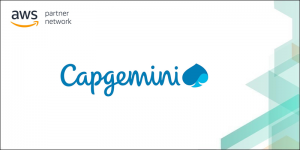 |
Capgemini |
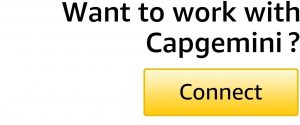 |
Mutual or insurance companies are often governed by members and have business and/or membership rules that govern membership grants. Such rules are classified as Inclusion rules and Exclusion rules.
Inclusion rules are business rules that reward customers who meet certain criterion, such as purchase of bonds or high-value financial instruments, and tenure or association with the company.
Conversely, Exclusion rules are defined to update/grant/revoke membership based on member activities, such as purchasing a certain products or product types, or having a secondary role on the product (particularly products that have joint holdings).
When implemented in legacy on-premises systems, such rules tend to be rigid in nature and impact business agility. Business users who depend on the IT department to make rule changes and updates triggered by changing business or regulatory requirements need to plan for development resource availability, change management, rules development lead times, and associated project costs.
This inhibits an organization’s ability to be agile and serve their members efficiently, expand memberships, and adapt quickly to changing business or regulatory requirements.
In this post, we will explore how Capgemini uses Amazon Web Services (AWS) to build a simple, agile, and configurable solution that implement business and membership rules on customer or master data. This is further improved using metadata to introduce or amend additional business rules.
Such a design pattern can be easily customized to additional business use case; not just customers or members.
Capgemini is an AWS Premier Consulting Partner and Managed Service Provider (MSP) with a multicultural team of 220,000 people in 40+ countries. Capgemini has more than 12,000 AWS accreditations and over 4,900 active AWS Certifications.
High-Level Architecture
The diagram below depicts the high-level architecture of a rules-based engine on AWS. The main components of this architecture are:
Rules repository that stores business rules.
Data store that hosts customer records or customer master.
Processing engine.
Data store to capture the results of the processing and maintain a member register.
End-to-end workflow orchestration mechanism.
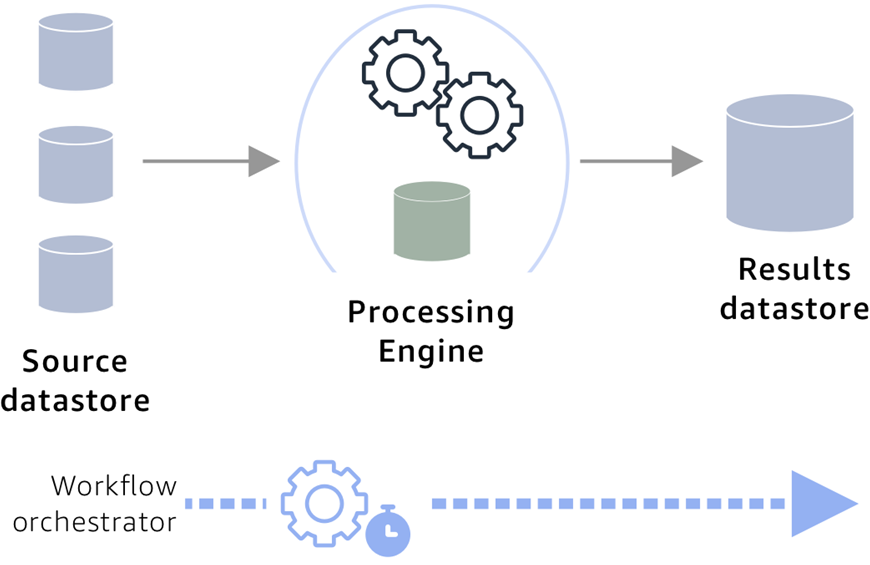
Figure 1 – High-level solution overview.
This architecture caters for cleaning and deduplicating customer data, and for creating master data where an organization may have multiple sources for customer data.
The rules engine by design is configurable, allowing business rules that can be configurable and maintained with rule-specific metadata for instance type of rule, category, and so on. The rules evaluation results are enriched and captured with details to maintain history to understand the member journey.
Figure 2 shows how such an architecture can be realized using AWS-native managed services that reduce spend on operational maintenance activities, such as patching and capacity management, while providing programmatic access to the service feature. This enables operational excellence via automation.
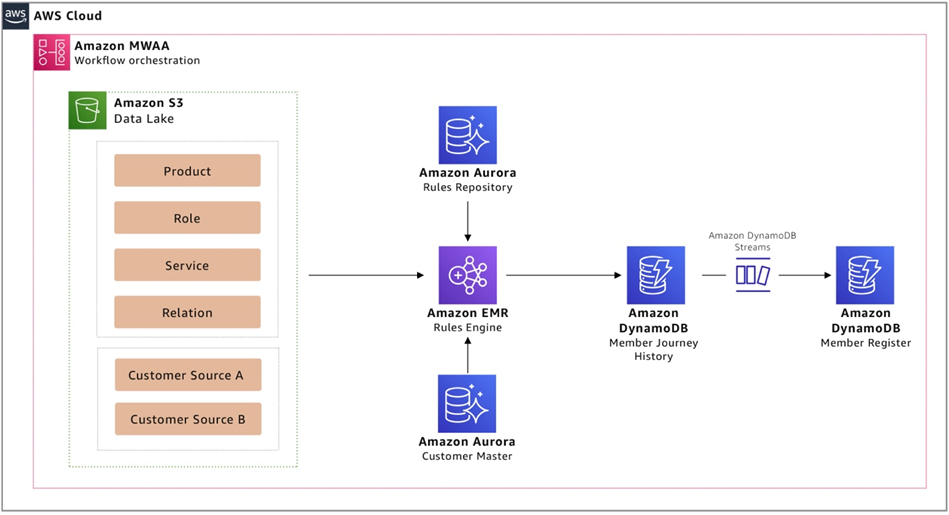
Figure 2 – Solution overview using AWS managed services.
AWS Implementation Solution Overview
The first step of implementing this solution is to get all of the customer and related data needed to run the business rules into your data lake. The data lake acts as the central location for all data sources on which the rules engine will run.
Additional important points to consider while implementing the data lake are:
Identify the critical data elements that would be required to run your business rules and the source systems that have these data elements.
If you have more than one customer data source, you can use a Master Data Management (MDM) tool like Informatica, Reltio, or other third-party tools to merge the customer data.
If there’s a golden source of customer data, this can be used for identification of memberships.
Ensure the customer data can be joined with other data elements like product, service, and roles so the business rules can be evaluated.
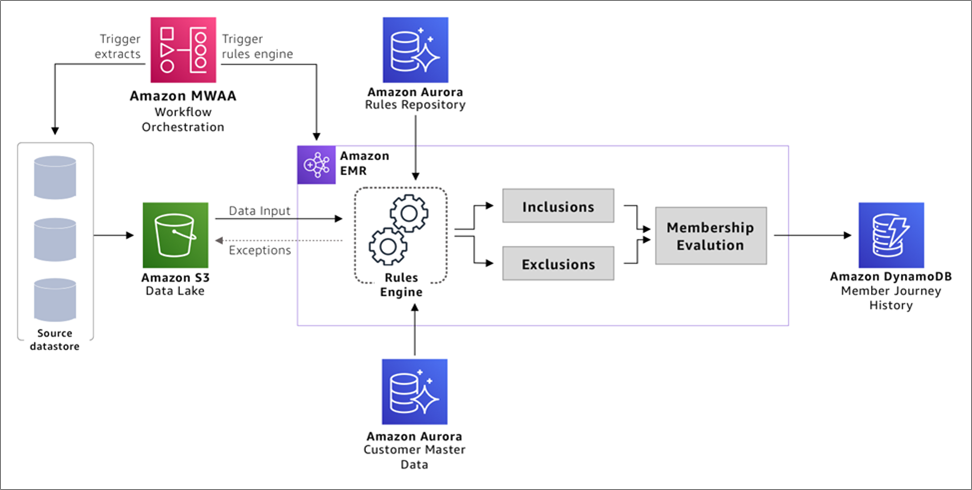
Figure 3 – Agile business rules engine implementation design on AWS.
The implementation uses the following AWS services:
Amazon Simple Storage Service (Amazon S3)
Amazon S3 is used to create a data lake of all data required for rules engine processing. As the data store aspect of the architecture, data lakes on S3 benefits from its 99.999999999% (11 nines) durability and object store nature, allowing the data lake to hold multiple data formats, datasets, and be able to be consumed by a variety of services in the AWS and third-party applications.
All customer information from the source environment is extracted and placed onto the data lake for subsequent processing by rules engine.
Amazon Aurora
Amazon Aurora is used to configure and capture the business rules with the metadata and reference data (like rule and membership types) that are needed to provision a simple relational database.
Aurora is a database engine for Amazon Relational Database Service (Amazon RDS) and meets requirements. This also provides a relational model that can be extended to include additional metadata like version of rule, active, or inactive rules.
These attributes make it easier to understand the customer/member journey and how and when they attained memberships, as well as which rules were in effect at that point in time and such.
Note that depending on the complexity of the rules and business requirements on how often these rules would change, you may want to configure the reference data accordingly.
Amazon EMR
Amazon EMR is used to create a data processer that can easily join data from different data stores and execute SQL in memory. It can also run rules in parallel, scale to changing business load, and be cost optimized by enabling transient features so you can save costs when the system is not in use.
Amazon EMR provides these features on AWS to rapidly process, analyze, and apply machine learning (ML) to big data using open-source frameworks.
The architecture uses Apache Spark on Amazon EMR due to its flexibility in being able to configure conditions and filters on the rules repository. Using SQL enables easier build and maintenance of rules.
Spark executes these SQL-based rules in parallel to identify the inclusions and exclusions against all of the customer data, and can be used to join with the other critical data elements (like product and service) and to validate against reference data configured in the rules repository.
Under the hood, the rules engine will:
Create data frames of the customer data from the data lake and rules repository.
Read the customer and critical data elements from the data lake and the rules and rule reference data from the Aurora database.
Distribute and run the inclusion or exclusion SQL rules in parallel against all of the customers to ensure every customer is evaluated against every rule.
Write the results from these SQL execution to an ephemeral storage or S3 bucket to be able to run evaluations based on the inclusions and exclusion results. This step can be executed in memory depending on your data volume and performance considerations.
Additional design considerations for the rules engine inclusions and exclusions modules:
Complexity: Depending on the complexity of the rules and rule categories, you may prefer to configure the complete SQL statements in the rules repository itself. The evaluation step takes into consideration all of the inclusions and exclusions for each customer. If a customer qualifies for inclusions, and depending on what exclusions apply, it will grant or disregard memberships.
Numeric inclusions and exclusions: To implement more complex business rules, the inclusion and exclusion rules can be granted numeric weights like 1, 2, 3 in the rule configuration when you design the rules repository. It would be a good idea to space the rule weights with multiples of 10 like 10, 20, 30.
Need to disregard: If there’s a need to disregard certain exclusions and instead override such exclusions when certain inclusion rules apply, these weights can be used in the evaluation step. Assign higher numeric weights to inclusion rules that override any exclusion rules. During evaluation, you can apply a sum function to all the weightages of each category; the aggregate of inclusion weights and exclusions weights can be used to grant or disregard memberships depending on whichever is higher.
The rules engine generates a set of customer inclusions and exclusions tagged with additional metadata like types of memberships. As a next step, these inclusions and exclusions are evaluated to determine a binary outcome that will tag only those customers that qualify under the various membership types.
Amazon DynamoDB
Amazon DynamoDB stores the results from the rules engine, as it provides a fully managed, serverless, key-value NoSQL database designed to run high-performance applications at any scale for end system consumption.
The membership records are is inserted into a DynamoDB database that maintains the history of results. The records can be inserted into DynamoDB using Python. This will be an insert only table to capture all the history.
Amazon DynamoDB can be partitioned on the master customer ID and sorted using a history created date. The benefit of partitioning the data on the same key used in the customer master makes it easier to combine these datasets easily. The history created date makes the sorting easier to generate the customer or membership journey.
Optionally, these results can be streamed using DynamoDB streams into another DynamoDB table to maintain a current member register with the latest details for easy access or current view of the member register. Often, businesses may have another key like membership number, so the benefit of using another current DynamoDB table with the current version is able to use an alternate partition key and enable faster access.
Amazon Managed Workflows for Apache Airflow (MWAA)
Amazon MWAA is used to orchestrate workflow sequencing of events required to ingest, transform, and load data using a managed service. This enables development teams to focus on defining the workflow sequences and not worry about the underlying infrastructure capacity and availability.
Conclusion
By using a combination of AWS managed services, Capgemini can build a cloud-based rules engine that can be scaled to increasing data volume, easily configured, and quickly accessed. This enables organizations to be completely agile in developing new business rules, or updating/retiring business rules, without relying on IT.
Capgemini, with its global experience, best-of-breed technology, process, and people, can partner with you to help design and build solutions that can be tailored to handle other medium to complex business rules for any industry, using AWS cloud-native services.
.
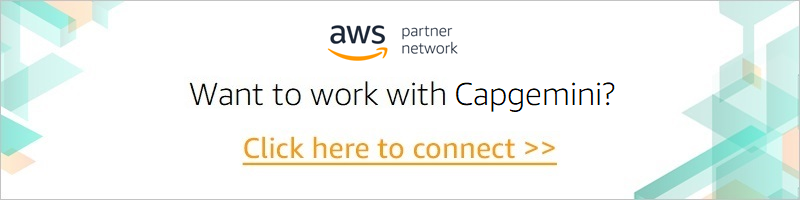
.
Capgemini – AWS Partner Spotlight
Capgemini is an AWS Premier Consulting Partner and MSP with a multicultural team of 220,000 people in 40+ countries. Capgemini has more than 12,000 AWS accreditations and over 4,900 active AWS Certifications.
Contact Capgemini | Partner Overview
*Already worked with Capgemini? Rate the Partner
*To review an AWS Partner, you must be a customer that has worked with them directly on a project.
Implementing Hyper-Personalization in the Banking Sector with Amazon EMR
=======================
By Bharani Subramaniam, Head of Technology – ThoughtWorks
By Mageswaran Muthukumar, Lead Consultant, Data Engineering – ThoughtWorks
By Nagesh Subrahmanyam, Partner Solutions Architect – AWS
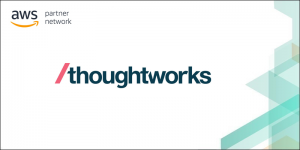 |
Thoughtworks |
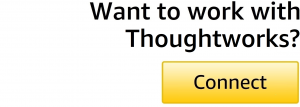 |
In the digital economy, customers expect immediate, relevant, and frictionless experience when they want to find, do, or buy something.
To meet these expectations, businesses need to deliver hyper-personalization for every one of their customers. This demands a comprehensive view of customers’ behavioral patterns across direct and indirect touchpoints.
Such a solution requires an elastic infrastructure, as you can’t predict the amount of compute and storage a solution will need up front. Moreover, a regulated industry like banking puts additional constraints on dealing with personally identifiable information (PII).
This post describes how ThoughtWorks, a global technology consultancy company, handled infrastructure and regulatory challenges and delivered a cloud-native, hyper-personalized data platform on top of Amazon EMR for a leading private sector bank in India.
ThoughtWorks is as AWS Competency Partner and leading global technology consultancy that enables enterprises and technology disruptors to thrive as modern digital businesses.
What is Hyper-Personalization?
Digital platforms are disrupting the traditional business models across many industries. Though there are a number of factors that make a digital platform successful, we have observed the strategy to sustain and promote usage of the platform is the one of most critical factors.
Traditionally, businesses focused most of their marketing efforts on acquiring new customers, but in the digital era they also need to continuously engage existing customers at the same time.
To achieve continuous engagement, some organizations have a dedicated “growth team” while others treat this as a part of their general marketing efforts. No matter how a business is set up, you need a good infrastructure to derive insights and make data-driven decisions.
Years of research point to the fact customers value contextual and personalized content over generic ads. Before we go further, let’s unpack the different levels of personalization and explore why hyper-personalization is so effective.
Assume your business has one million consumers and your marketing department wants to promote 10 offers, each with 10 variants, to keep these customers engaged.
Though there can be many ways to achieve this, we can broadly categorize communication mechanisms into three distinct categories, as shown in the levels of personalization chart below: generic messaging, segmentation, and hyper-personalization. The effectiveness of communication increases as the focus of personalization increases.
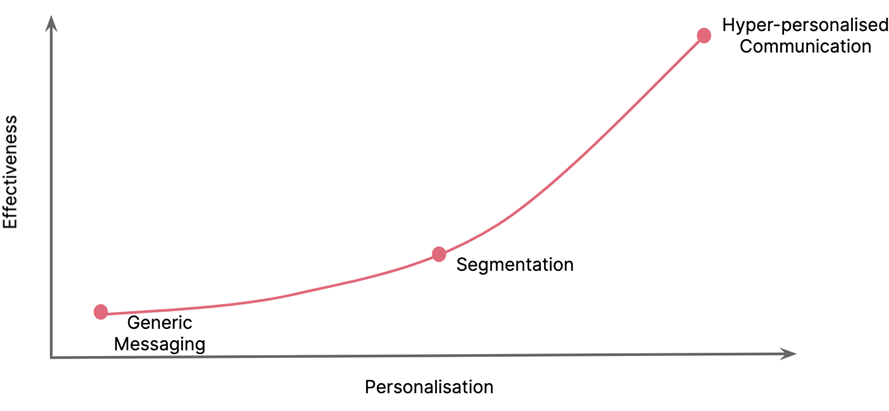
Figure 1 -Levels of personalization.
The following sections describe the levels of personalization.
Generic Messaging
In this approach, you would simply send all 100 offers (10 offers with 10 variants) to every one of your million customers. You may overlay the customer name or gender to make it look personalized, but this kind of generic messaging is mostly ineffective and there’s a high probability your customers would treat it as spam.
Segmentation
In this approach, you’d leverage the knowledge gained about your customers through their purchase history and preferences to effectively cluster or segment them into distinct personas. You can also filter the offers according to each segment. Segmentation leads to better conversion rate as it’s more targeted than generic messaging.
Hyper-Personalization
With hyper-personalization, you would go a step further than just segmenting your user base. You don’t send the offers at random intervals, but rather watch for signals from customer behavior and promote valid offers that address the needs of your customer in a timely manner.
Hyper-personalization is far more effective than other approaches, but it does require sufficient data to infer behavioral patterns and the ability to process them in near real-time.
Limitations with On-Premises Infrastructure
When we analyzed the volume and nature of transactional data required to create the behavioral model of our customers, we realized a few key insights:
Daily volume of raw input data across all sources is approximately 1 TB.
Volume of the output (customer model and valid offers) is approximately 10 GB, or one tenth of the input volume.
When we compute the customer model with thousands of attributes across different stages in our data pipeline, the data volume expands up to 5x the input volume for the cluster.
With this insight, it was clear we can’t depend only on the on-premises data center capacity; rather, we need a hybrid-cloud model. For security considerations, the data preparation and sanitization steps are executed from on-premises data centers.
ThoughtWorks decided to leverage AWS for the big data pipeline, and this hybrid cloud approach enabled them to focus on quickly testing business hypotheses rather than operating the big data infrastructure.
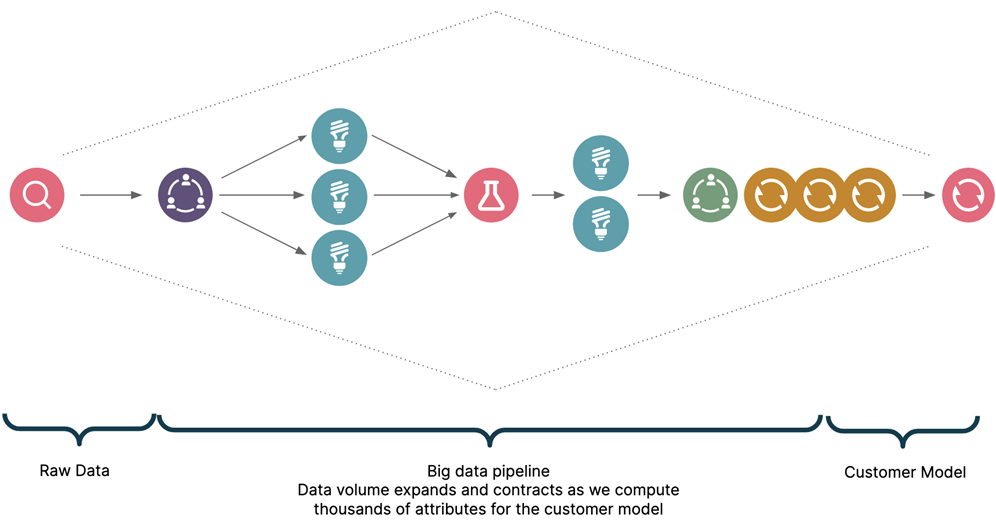
Figure 2 – Expand and contract nature of data.
Hybrid Cloud Approach Using Amazon EMR
The following table describes our approach at a high level.
No. |
Stages |
Where |
Descriptions |
1 |
Integrate with system of records (SoRs) |
On-premises |
This layer is responsible for integrating with hundreds of internal systems via file, Confluent Kafka, or API interfaces. |
2 |
PII scrubber |
On-premises |
Once we have the data, we scrub all PII details which aren’t required for the data platform running on the cloud. |
3 |
Tokenization and encryption |
On-premises |
Tokenize the record to protect the remaining PII information. Encrypt the reverse lookup map and store it in a RDBMS on the on-premises data center. |
4 |
Big data processing |
AWS Cloud |
We leverage Apache Spark in Amazon EMR clusters to compute thousands of metrics to effectively model the customer behavior. |
5 |
De-tokenize and delivery |
On-premises |
At this stage, we observe patterns in user behavior and trigger the communication process based on the model we have computed from the cloud. De-tokenization happens just-in-time before communication delivery. |
To illustrate the five stages of hyper-personalization as described in the table, let’s consider a fictional user named Saanvi Sarkar and see how the model gets progressively built as we go through each stage.
Integration with System of Records (SoRs)
We carefully mapped our requirements with hundreds of data sources from within and outside the bank. Details on such integrations and mapping of data sources are beyond the scope of this post.
In this stage, we’d have all transactional details of Saanvi Sarkar from their existing banking account. We also have access to credit and other scoring details from regulatory sources.
Personally Identifiable Information (PII) Scrubber
The first stage in the privacy preserving layer of the solution is the PII scrubber. Depending on the nature of the attributes, we apply a few strategies to protect the end user’s privacy:
Any PII attribute that’s not required in later stages of processing is scrubbed, meaning it’s completely dropped from our dataset.
Some attributes, like age, are transformed from absolute values to ranges without any provision for reverse lookup. Example: 23yr to 20-25yrs.
A few other attributes, like external references, are partially masked.
At this stage, Saanvi Sarkar details look like this:
<Key Identifier> <Necessary PII Data> <Non-PII Data>
Tokenization and Encryption
In the next stage, we tokenize the key identifier so that even if there is a breach it will be difficult for bad actors to make sense of the records.
<Key Identifier> is tokenized and substituted with random values. The reverse lookup is stored in a secured system in the on-premises data center.
<PII Data> like email and phone numbers are removed from the record, and encrypted and stored separately for later use during offer delivery.
After tokenization and encryption, the output record of Saanvi Sarkar will look like <Token>< Non-PII Data>. At this stage, the data is anonymized and safe for processing in the cloud.
Big Data Processing in Amazon EMR
The bulk of the data processing happens in this stage. Cleansed data that’s safe for computation in the cloud is fed into data pipelines in Amazon EMR Spark clusters.
We have a mix of batch and streaming workloads as described in the architecture diagram in Figure 3 below. The entire pipeline can be broken down into two stages: modelling customer behavior, and the matching engine.
Modelling Customer Behavior
Data arrives either via Kafka topics or via files from Amazon Simple Storage Service (Amazon S3). We utilize Apache Airflow to schedule jobs in Amazon EMR clusters.
Since we have hundreds of data sources, we have to support both continuous streams of updates and batches arriving in hourly, daily, or even weekly intervals.
Apache Airflow orchestrates all the periodic batch jobs. Once triggered, these jobs create ephemeral Amazon EMR Spark clusters to incrementally compute the customer model.
For streaming use cases, we utilize Spark Streaming in Amazon EMR to process real-time continuous updates from Kafka topics.
To support ad hoc exploration, we use AWS Glue to effectively catalog files in S3 and create necessary schema for Amazon Athena.
We can now infer that Saanvi Sarkar regularly deposits ₹50,000 within the first two weeks of every month and their average balance never went below ₹300,000. We also know she extensively travels by two wheeler based on fuel expenses.
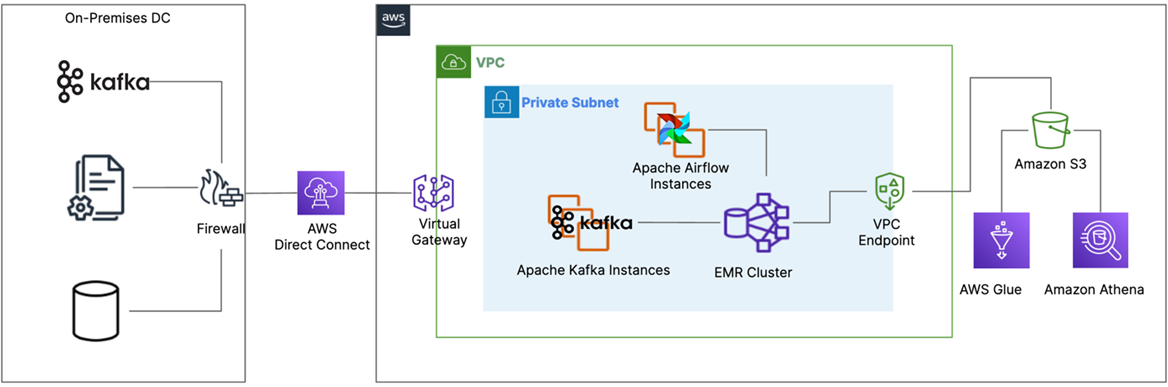
Figure 3 – Hyper-personalization architecture.
Matching Engine
We wanted a flexible way to express the changing needs of the marketing team, so we created a custom domain specific language (DSL) that’s easy to understand but at the same time expressive enough to capture the changing needs.
The DSL takes the customer model, inventory of offers along with its variants, and the real-time behavioral data to effectively match suitable offers.
Each offer and its variants are tagged with potential eligibility criteria, like what channels of communication are applicable and what conditions must be met in the customer model.
The DSL also takes customers’ preferences into consideration. For example, if they have opted out of a channel or types of promotions or prefer certain days of the week.
We designed the DSL to be flexible and extensible enough to express different constraints and chain actions together. An example of this is sending a special offer if the customer has positively responded to the previous one.
Users from the campaign management team continuously come up with offers and variants and capture the matching logic in the DSL. Once executed, the DSL takes care of creating necessary jobs in Airflow, which in turn orchestrates the matching pipelines in Amazon EMR Spark clusters.
In this example, there is a high probability that Saanvi Sarkar is looking to buy a car. Given this context, the matching engine will pick the car loan offer with attractive interest percentage based on Saanvi Sarkar’s credit history.
De-Tokenize and Deliver
The matching engine communicates the match as <Token> <Offer> to the on-premises data center. Since the data in the cloud is tokenized for protecting customer privacy, we need to de-tokenize before delivering the offer.
For the given <Token>, we retrieve the actual customer identifier. This is handled securely by the tokenization system. Output of this stage looks like this: <Key Identifier> <Offer>.
Once we know the <Key Identifier> of the customer, we need to retrieve additional information based on the channel, such as the email or phone number to send the offer to. Since these are PII data, it will be encrypted during the tokenization stage. We have to decrypt to get these details. Output of this stage looks like this: <Key Identifier> <Necessary PII Data> <Offer>.
With <Key Identifier> <Necessary PII Data> <Offer> we send the offer to appropriate delivery systems via push notifications, email gateway, or SMS providers.
The matching engine predicted the need of the Saanvi Sarkar to own a car and came up the relevant offer for the car loan with customized interest rate based on credit history. This approach significantly transformed the customer relationships for the bank.
Instead of designing a group of offers and sending them to millions at a time, the bank offers immediate, relevant, and frictionless experiences to its diverse customers.
Summary
Digitally savvy customers want to feel delighted and they expect organizations to add value to their lives with relevant and timely information. ThoughtWorks and AWS have seen hyper-personalization help in shifting the focus from static promotions to address the dynamic needs of their customers.
From our experience, some key factors to consider while implementing hyper-personalization initiatives are:
Come up with a strategy to keep customers engaged. If users are not active, the benefits of a digital platform erode over time.
Constantly revisit the number of data sources required to effectively build customer profile. Too much accuracy in personalization may have a negative effect as some customers may find your solution to be invading their privacy.
Devise a flexible mechanism to capture the moving needs of the marketing team. ThoughtWorks built a custom domain specific to declaratively capture the details of the offer without exposing the complexity of underlying implementation.
Understand the regulatory implications before designing the architecture. Some parts may still be required to be on-premises data center.
Leverage cloud for scaling big data infrastructure. ThoughtWorks observed a 5x increase in data volume during transformations and used Amazon EMR for scaling ad hoc experimentations and running the production workload.
If you are interested in knowing more about hyper-personalization, here’s a good collection of articles addressing the dynamic micro-moments of customers.
If you’re interested in the implementation details of streaming processing using Apache Spark and Apache Kafka, learn more in this AWS Big Data blog post.
.
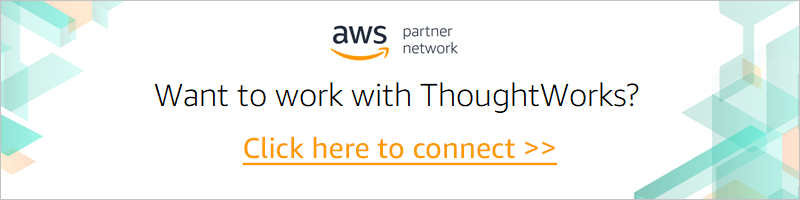
.
ThoughtWorks – AWS Partner Spotlight
ThoughtWorks is an AWS Competency Partner and leading global technology consultancy that enables enterprises and technology disruptors to thrive as modern digital businesses.
Contact ThoughtWorks | Partner Overview
*Already worked with ThoughtWorks? Rate the Partner
*To review an AWS Partner, you must be a customer that has worked with them directly on a project.
Creating Unique Customer Experiences with Capgemini’s Next-Gen Customer Intelligence Platforms
=======================
By Deepika Giri, Sr. Director, Insights and Data – Capgemini
By Vikas Nambiar, Manager, Partner Solutions Architect – AWS
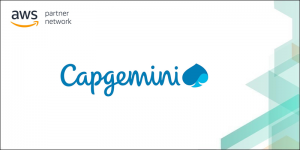 |
Capgemini |
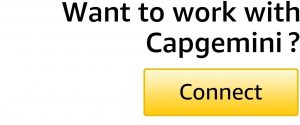 |
As organizations evolve and embrace digital transformation, they realize customer value delivered is a function of quality of engagement through various channels: digital, online, and in-person.
Customer experience is at its best when a customer perceives the experience offered is unique and aligns to their preferences. The need to engage, at a very personal level, becomes key.
Organizations have realized that knowledge of data around customer behaviors are crucial for a differentiated customer experience.
Traditionally, customer relationship management (CRM) tools were considered the mainstay for first-party customer data, whereas now there are a plethora of solutions to choose from.
This ranges from a standalone tool or service to a complete ecosystem that deliver superior customer experience platforms, including customer data platforms (CDP) and marketing data management platforms (DMP), to name a few.
Businesses, meanwhile, have realized there is no single product or service that provides all of the features, and building such a platform requires a complex process. A robust and reliable customer platform has to integrate and aggregate first-, second- and third-party data seamlessly and in real-time in a cookie-less paradigm.
In this post, we’ll look at how Capgemini’s data and analytics practice implements Customer Intelligence Platforms on Amazon Web Services (AWS) to help companies a unified data hub. The platforms enable organizations to convert customer data into insights that can be used for reporting, building artificial intelligence (AI) and machine learning (ML) predictive analytics capabilities, and more.
Capgemini is an AWS Premier Consulting Partner and Managed Service Provider (MSP) with a multicultural team of 220,000 people in 40+ countries. Capgemini has more than 12,000 AWS accreditations and over 4,900 active AWS Certifications.
Capgemini Reference Architecture
Capgemini has worked with clients globally, across multiple industry sectors, and helped customers in their data transformation journey. This rich and deep industry experience and best practice knowledge has helped Capgemini simplify and industrialize the approach of building a modern data estate, designed to deliver business value and be future proof.
The Capgemini reference architecture outlined in this post serves as a blueprint to build next-generation data platforms that are robust, scalable, and drive innovation. This isn’t just a technology platform but a framework that brings together people, processes, and technology. The architecture covers all aspects of data, from ingestion to consumption.
Capgemini’s reference architecture is designed to be modular and decoupled, covering all major aspects of a data platform including:
Platform setup and management covering cloud platform planning and setup in addition to security aspects.
Data governance includes data quality, privacy, lineage, metadata management, and archival aspects.
Data foundation includes ingestion, both real-time and batch, data transformation, processing, and curation.
Advanced analytics foundations include data preparation and wrangle, model development, deployment and testing.
AI/ML includes custom AI and cognitive application deployment.
Visualization includes business intelligence (BI) reporting and self-service capabilities exposed via API interfaces.
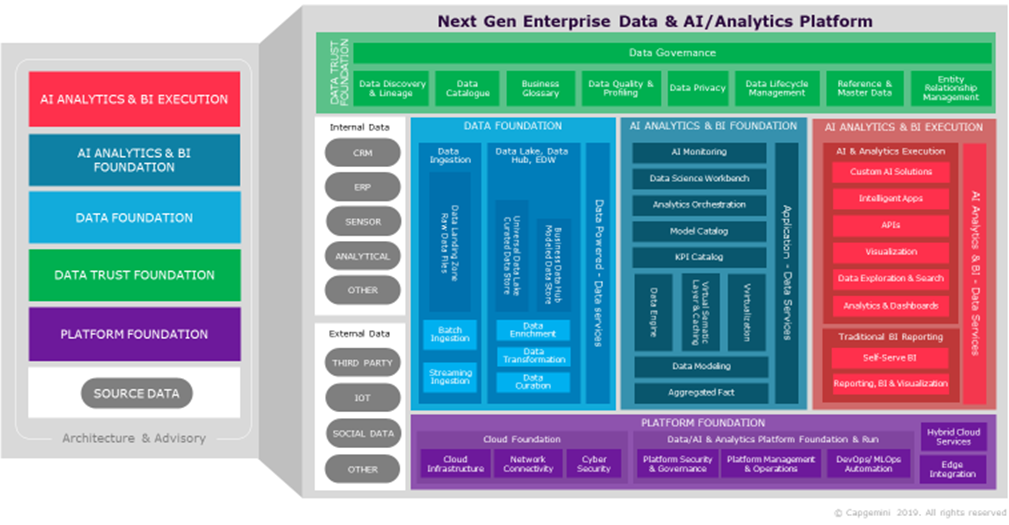
Figure 1 – Capgemini customer data and AI platform reference architecture.
Customer Experience Technology Ecosystem View
Given the need to create a differentiated customer experience, major CRM system vendors have built systems with capabilities to integrate customer data. The goal is to help drive insights and activation to marketing and sales, customer care, and ecommerce systems.
There are many customer data platforms that serve to integrate all customer data and enrich them through AI/ML. These share actions to be delivered via marketing data management platforms or existing customer marketing or sales platforms.
However, the data capabilities of such implementations can be limiting, albeit they enable organizations to build a unified customer profile.
Such implementations are incomplete without the capability to provide a unique enterprise-wide customer ID that is derived from all parts of customer data spread across their applications. In addition, they are not specialized in data quality and privacy, golden record generation, and management and sector specific solutions.
This implementation also needs to be further integrated with customer behavior data to generate consumable products such as customer segmentation, propensity models, cognitive services and measurements, and insights.
Thus, Capgemini’s Customer Intelligence Platform on AWS serves as a robust and reliable source of harmonized customer-related data.
A great degree of personalization is possible when all behavior and customer knowledge is deep and complete. A large variety of use cases can be built around this design using the customer intelligence gathered.
Below are some of the relevant use cases:
Predictive analytics: Customer churn prediction by integrating data from CRM and loyalty systems; creating a customer score to predict customer satisfaction levels; decreasing customer spend or lifetime value of long-standing customers can indicate an unhappy customer.
Real-time streaming analytics: Next Best Action/Next Best Offer using customer purchase history, customer segment, and browsing behaviors to define the right product or service offer; irrelevant offers negatively impact an organization’s branding.
Descriptive analytics: Lifetime value of a customer based on tenure and spending patterns is done using predictive AI/ML models; this information can drive marketing campaigns across digital and non-digital channels.
Target Architecture for a Customer Intelligence Platforms
Capgemini’s Customer Intelligence Platforms on AWS enable superior omnichannel customer experiences, empowering businesses to be innovative, agile, and pioneers in the way they operate. The platforms serve as a foundational data layer that delivers robust customer data and insights to applications like marketing, sales, customer care, and ecommerce.
Please note that the following architecture addresses common data ingestion, curation, and analytics foundation aspects of a typical solution. This can be further expanded to include platform and data governance aspects based on an organization’s operational requirements.
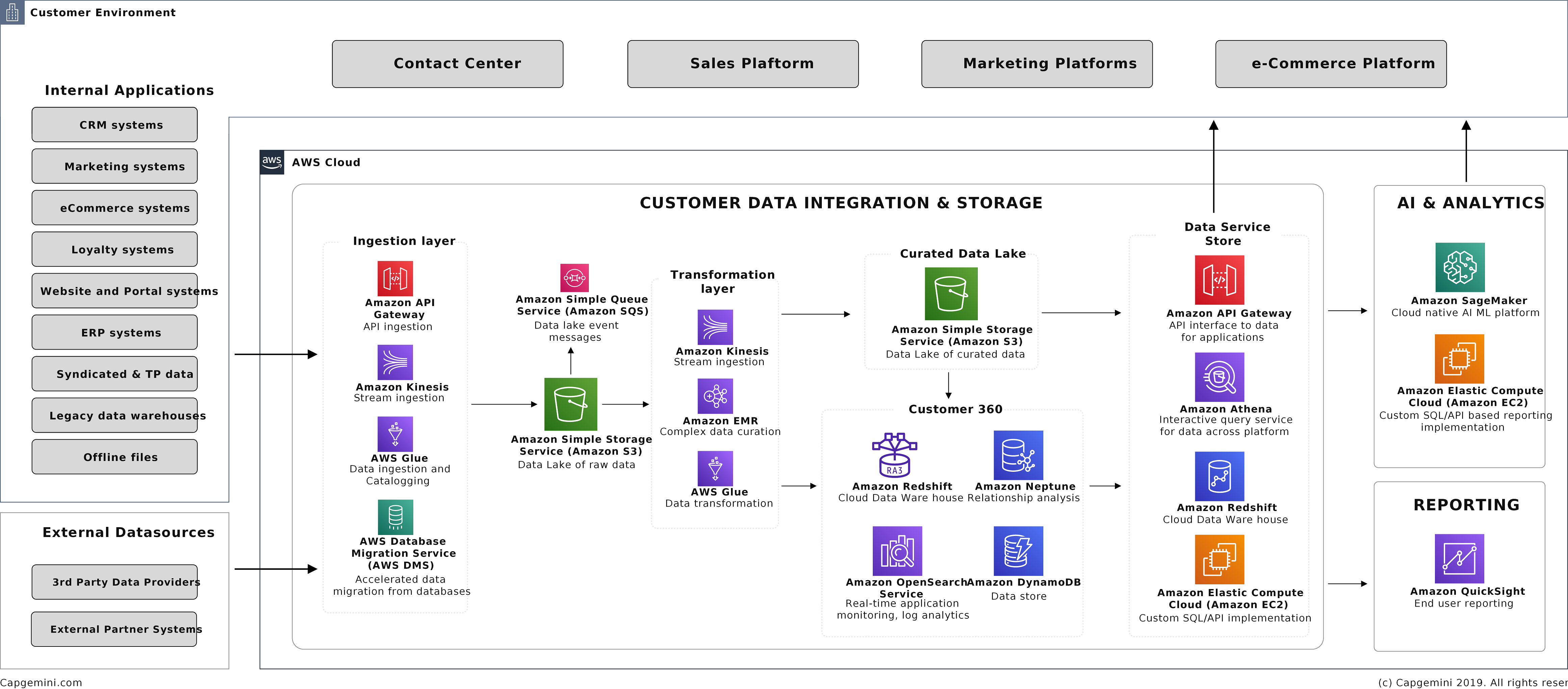
Figure 2 – Capgemini customer data and AI platform implementation design overview.
Key AWS services leveraged in the architecture:
AWS Glue: This is a great tool for batch ingestion of large volumes of data form sources. Capgemini can also set up rules for data profiling and cleansing on AWS Glue pipelines through Amazon S3 buckets.
Amazon Kinesis: This is used for real-time and streaming data ingestion into the data platform.
Amazon S3: This serves as the landing zone for all data from CRM, POS, loyalty, online, ecommerce, social media, and email. In addition, S3 will be the curated data lake layer that serves as the input zone for all data science and advanced analytics solutions to be built around the customer data.
Amazon EMR: This helps perform certain complex transformation of the data ingested into the platform.
Amazon Redshift: All of the aggregated customer 360 profiles will be stored on the Amazon Redshift data warehouse.
Amazon Redshift Spectrum: This allows data from the curated S3 landing zone to be accessed using a simple queries for analysis.
Amazon SageMaker: This is leveraged to build and train ML models using the data in the S3 curated layer. These models are deployed, and the outputs are either fed to dashboards or downstream applications via API integration or other AWS services.
AI cognitive services: All cognitive AI capabilities may be leveraged to build machine learning solutions, aligned to a customer environment, and requirements.
Amazon Forecast: This service allows organizations to build a time series-based forecasting model for sales, consumptions, and more.
Amazon Lex: This service allows companies to build conversational AI interfaces that help them connect with end users and customers via meaningful and contextualized interactions.
Amazon QuickSight: This service helps publish dashboards using the insights around customer intelligence data available on the platform.
Conclusion
In the current digital environment, without a unified data hub that converts customer data into insights, an organization’s capability to retain their customers will be impacted.
Such a unified data hub, enriched with AI/ML services implemented to requirements, provides improved and unique customer experiences that are aligned to their interests and preferences. Platforms that achieve this can increase return customer numbers and deliver higher customer satisfaction numbers.
Capgemini’s data and analytics practice, along with their Customer Intelligence Platforms on AWS, global experience, best-of-breed technology, process, and people can help enterprises create differentiated customer experiences.
Learn more about how Capgemini is turning customer value analytics into insights, decisions, and business value.
.
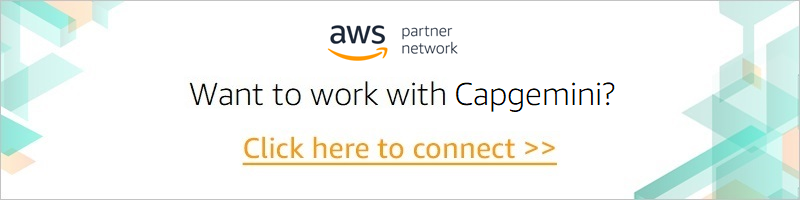
.
Capgemini – AWS Partner Spotlight
Capgemini is an AWS Premier Consulting Partner and MSP with a multicultural team of 220,000 people in 40+ countries. Capgemini has more than 12,000 AWS accreditations and over 4,900 active AWS Certifications.
Contact Capgemini | Partner Overview
*Already worked with Capgemini? Rate the Partner
*To review an AWS Partner, you must be a customer that has worked with them directly on a project.
Managing Machine Learning Workloads Using Kubeflow on AWS with D2iQ Kaptain
=======================
By Kiran Anna, Sr. Product Manager – D2iQ
By Young Jeong, Partner Solutions Architect – AWS
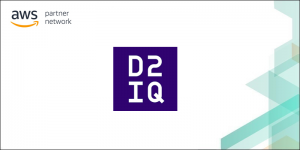 |
D2iQ |
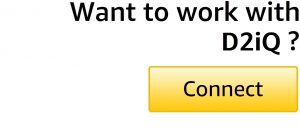 |
While the global spend on artificial intelligence (AI) and machine learning (ML) was $50 billion in 2020 and is expected to increase to $110 billion by 2024 per an IDC report, AI/ML success has been hard to come by—and often slow to arrive when it does.
87% of AI/ML initiatives never make it to production (VentureBeat, July 2019).
55% of organizations have not deployed a single ML model to production (Algorithmia, December 2019).
50% of “successful” initiatives take up to 90 days to deploy, with 10% taking longer than that (Algorithmia, December 2019).
In many enterprises, even just provisioning the software required can take weeks or months, further increasing delays.
There are four main impediments to successful adoption of AI/ML in the cloud-native enterprise:
Novelty: Cloud-native ML technologies have only been developed in the last five years.
Complexity: There are lots of cloud-native and ML/AI tools on the market.
Integration: Only a small percentage of production ML systems are model code; the rest is glue code needed to make the overall process repeatable, reliable, and resilient.
Security: Data privacy and security are often afterthoughts during the process of model creation but are critical in production.
Kubernetes would seem to be an ideal way to address some of the obstacles to getting AI/ML workloads into production. It is inherently scalable, which suits the varying capacity requirements of training, tuning, and deploying models.
Kubernetes is also hardware agnostic and can work across a wide range of infrastructure platforms, and Kubeflow—the self-described ML toolkit for Kubernetes—provides a Kubernetes-native platform for developing and deploying ML systems.
Unfortunately, Kubernetes can introduce complexities as well, particularly for data scientists and data engineers who may not have the bandwidth or desire to learn how to manage it. Kubeflow has its own challenges, too, including difficulties with installation and with integrating its loosely-coupled components, as well as poor documentation.
In this post, we’ll discuss how D2iQ Kaptain on Amazon Web Services (AWS) directly addresses the challenges of moving machine learning workloads into production, the steep learning curve for Kubernetes, and the particular difficulties Kubeflow can introduce.
Introducing D2iQ Kaptain
D2iQ is an AWS Containers Competency Partner, and D2iQ Kaptain enables organizations to develop and deploy machine learning workloads at scale. It satisfies the organization’s security and compliance requirements, thus minimizing operational friction and meeting the needs of all teams involved in a successful ML project.
Kaptain is an end-to-end ML platform built for security, scale, and speed. With some of its core functionalities leveraged from Kubeflow, Kaptain supports multi-tenancy with fine-grained role-based access control (RBAC), as well as authentication, authorization, and end-to-end encryption with Dex and Istio.
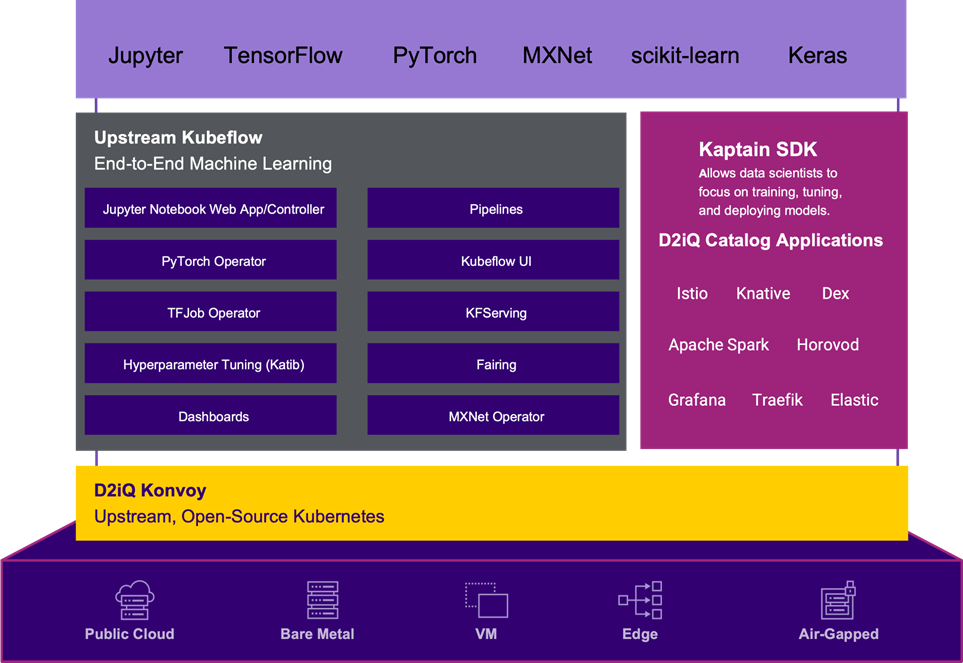
Figure 1 – Kaptain architecture.
Kaptain has many components that are geared towards development of ML models. The platform provides a Python-native user experience, although Spark support is available through Scala with Apache Toree.
The Jupyter Notebooks that many data scientists use for ML projects are pre-installed with the following:
Libraries: Seaborn, statsmodels, SciPy, Keras, scikit-learn, PySpark (for ETL and ML), gensim, NLTK, and spaCy.
ML workflow tools: Spark and Horovod for distributed training and building data pipelines, parameter tuning, deployment of models with auto scaling.
Frameworks: Fully tested, pre-configured images for TensorFlow, PyTorch, and MXNet, with CPU or GPU support.
Challenges of ML Adoption in the Cloud-Native Enterprise
While setting up a Jupyter notebook on a single laptop is easy, it becomes tricky with custom libraries, drivers while provisioning hardware, or with demands for portability, security profiles, service accounts, credentials, and so on. In short, notebooks are not that simple to manage in an enterprise environment.
On top of providing a familiar notebook environment, Kaptain provides easy steps to create a full ML workload lifecycle. The Kaptain SDK enables data scientists to perform distributed training on a cluster’s resources, conduct experiments with parallel trials for hyperparameter tuning, and deploy the models as auto-scaling web services with load balancers, canary deployments, and monitoring.
The software development kit abstracts away the production tasks and allows data scientists to focus solely on model production.
Take, as an example, a data scientist who wants to tune the hyperparameters of a model by running four experiments in parallel, with each experiment running on two machines.
The steps to approach this on Kubernetes without the Kaptain SDK are:
Write the model code inside a notebook, or the preferred development environment.
Build a Docker image of the model and its dependencies outside of a notebook, locally or through CI/CD (necessitating a context switch).
Define the manifest for Katib for model tuning, which consists of both a Katib-specific specification but also one for the job itself, based on the framework used (another context switch).
Deploy the experiment from the command line with the Kubernetes cluster properly set up on the local machine (another context switch).
Monitor the logs to see when the experiment is done.
Extract the best trial’s model from the logs.
Push that model to the registry.
With the Kaptain SDK, the process is far simpler and more data scientist friendly. The Kaptain SDK allows data scientists to do all of these steps from Python without having to do more than Step 1 above.
Here is how simple that process looks as Python code:
from kaptain.model.models import Model
from kaptain.hyperparameter.domains import Double, Discrete
model = Model(
id="my-awesome-model",
main_file=trainer.py, ...)
hparams = {
"--learning-rate": Double(0.2, 0.8),
"--epochs": Integer(10, 100)
}
model.tune(
workers=2,
parallel_trials=4,
hyperparameters=hparams,
objectives=["accuracy"])
The open-source edition of Kubeflow has well over 50 components, integrations, and related initiatives, which can make life as a data scientist confusing. How do you choose from a set of tools you’re not that familiar with?
D2iQ ensures the best cloud-native tools are included in Kaptain, and only those that provide unique functionality that makes sense for enterprise data science use cases.
Through research and review, evaluation is done on a set of core criteria, including:
Capabilities vs. requirements
Codebase health
Community activity and support
Corporate or institutional backing
Project maturity
Roadmap and vision
Overall popularity and adoption within the industry
D2iQ regularly runs tests with mixed workloads on large clusters to simulate realistic enterprise environments. This ensures the entire stack is guaranteed to work and scale. Each release of Kaptain is also “soaked,” or run with a heavy load for a certain duration, to validate the performance and stability of the system. Kaptain can be updated with zero downtime.
Do-it-yourself (DIY) machine learning platforms, with so many moving parts, can easily expose your business to unnecessary security risks. In June 2020, ZDNet reported a widespread attack against DIY Kubeflow clusters that failed to implement proper security protocols. Kaptain is protected from such vulnerabilities by design, and is deployed exclusively behind strict authentication and authorization mechanisms.
All of these factors combine to reduce the complexity and friction associated with running ML workloads on AWS. By simplifying the process of getting ML workloads into production, Kaptain enables organizations to make greater use of this game-changing technology.
Customer Success Story
One of D2iQ’s customers is a government scientific research organization that addresses a broad range of technical and policy questions. Many of the organization’s data scientists work on AWS, but much of the enormous volumes of data generated by the organization are on edge locations.
The costs of moving this data from the edge to the center of the network, or from one region to another, were quickly becoming prohibitive. Data scientists were frustrated by the long lag time between requesting IT resources and actually receiving them.
Data scientists also had to manage their own tech stack, resulting in a lack of repeatable standards. From the IT team’s perspective, lack of governance for deployed workloads led to concerns around security and inefficient use of resources.
The organization quickly adopted D2iQ Kaptain upon its introduction. Kaptain runs as a workload on the D2iQ Konvoy Kubernetes distribution, which was deployed on AWS and the customer’s data centers.
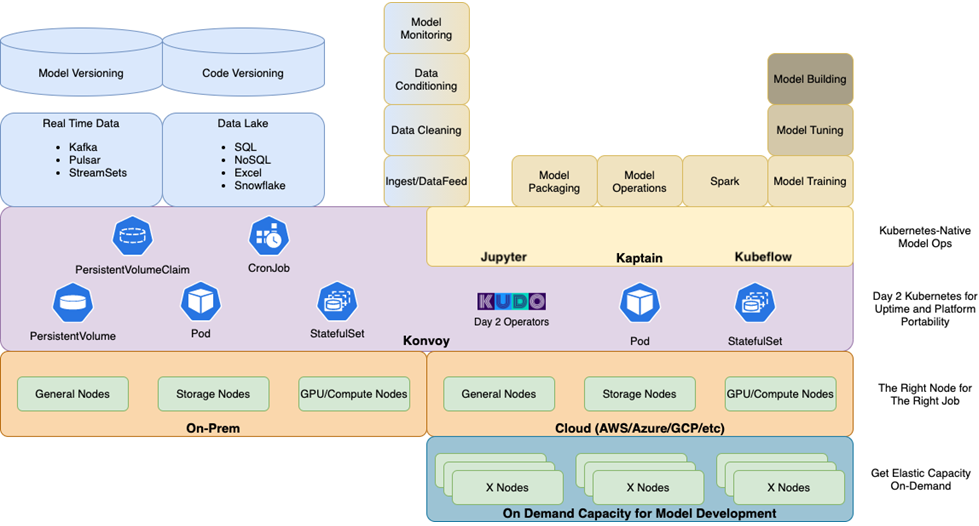
Figure 2 – Kaptain deployment reference architecture.
Kaptain solved three big problems for the organization:
First, Kaptain provided data scientists with a notebooks-first approach to their work. This insulated them from the complexities of the underlying infrastructure, thus saving time and improving their productivity.
Second, Kaptain allowed the customer’s IT team to provide internal data scientists with an ML platform as a service, cutting the time required to provision infrastructure from days to hours. The infrastructure-agnostic portability and scalability of Kubernetes enabled the IT team to place workloads where it made the most sense for them, on AWS or in their data centers.
Finally, because Kaptain (and its Kubernetes underpinnings) works the same everywhere and provides a uniform user experience irrespective of the underlying platform, types of work affected by data gravity (for example, training models on huge data sets) could be done where they made the most sense.
Plus, D2iQ’s expert support and training services extend the reach of the organization’s IT team and help them maximize the satisfaction of their internal data scientist and data engineer customers.
Conclusion
D2iQ Kaptain enables data scientists and data engineers to harness the scalability and flexibility of Kubernetes without having to struggle with its complexity.
D2iQ Kaptain and the Kaptain SDK provide a notebooks-first approach to managing the entire machine learning lifecycle, from model development to training, deployment, tuning, and maintenance.
By reducing the complexity and friction of getting ML models from development to production, Kaptain helps organizations increase the share of models actually being implemented and providing positive returns on investment.
To learn more about D2iQ Kaptain, see a demo, or arrange a free trial, please visit the Kaptain website.
.
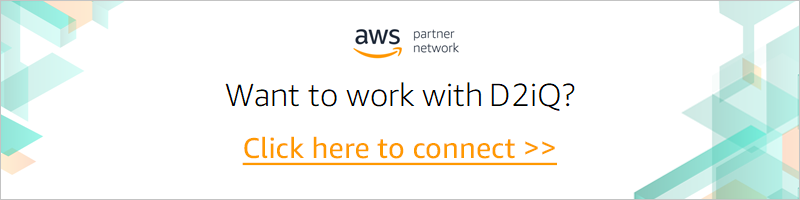
.
D2iQ – AWS Partner Spotlight
D2iQ is an AWS Containers Competency Partner that delivers a leading independent platform for enterprise-grade Kubernetes.
Contact D2iQ | Partner Overview
*Already worked with D2iQ? Rate the Partner
*To review an AWS Partner, you must be a customer that has worked with them directly on a project.
Page 1|Page 2|Page 3|Page 4